Real-Time Irish Transit Analytics
Apache NiFi, Postgresql, GenAI, Apache Kafka, Apache Flink, JSON, GTFS
Let’s hop on a bus in Ireland!
We need to load static (rarely changing lookup data). We can do this with NiFi very easily. We build and insert these into new Postgresql tables.
See me here:
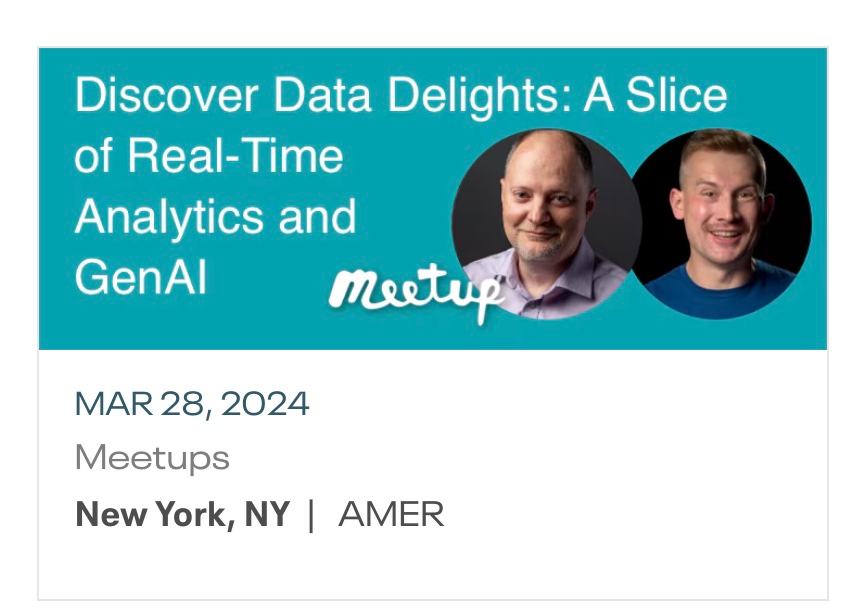
ChatGPT Authored Introduction:
Unlocking the Future of Transportation: Real-Time Irish Transit Analytics
In the bustling landscape of modern transportation, the ability to harness real-time data is not just a competitive advantage; it’s a necessity. In Ireland, where efficient transit systems are the lifeblood of daily commutes and city connectivity, the fusion of cutting-edge technologies is revolutionizing how we understand and optimize public transportation. This article delves into the world of Real-Time Irish Transit Analytics, where Apache NiFi, PostgreSQL, GenAI, Apache Kafka, Apache Flink, JSON, and GTFS converge to create a dynamic and responsive ecosystem.
Every day, thousands of passengers rely on Ireland’s public transit systems to navigate cities, reach work, or simply explore the beauty of the countryside. Yet, behind the scenes of this seemingly seamless operation lies a complex network of data streams, from vehicle locations to passenger counts, schedules to service updates. Here, Apache NiFi emerges as a pivotal tool, seamlessly orchestrating the flow of data from various sources into a unified pipeline.
PostgreSQL steps in as the reliable database backbone, providing a robust foundation for storing and querying vast amounts of transit data. With the power of GenAI, machine learning algorithms sift through this data trove, uncovering valuable insights into passenger behaviors, traffic patterns, and optimal routes.
But data is only as valuable as its timeliness, and this is where Apache Kafka and Apache Flink shine. Kafka acts as the real-time messaging hub, ensuring that updates from buses, trains, and stations are instantly propagated through the system. Flink’s stream processing capabilities then come into play, analyzing incoming data on the fly to generate actionable intelligence.
In the realm of data interchange, JSON (JavaScript Object Notation) emerges as the lingua franca, facilitating seamless communication between different components of the analytics ecosystem. And anchoring it all is the General Transit Feed Specification (GTFS), a standardized format for public transit schedules and geographic information, ensuring interoperability and accuracy across the board.
Join us on a journey through the intricacies of Real-Time Irish Transit Analytics, where these technologies converge to enhance efficiency, improve passenger experiences, and pave the way for the future of smart transportation.
An important source of data is the static GTFS lookup tables provided a zip file of CSV. We can download and parse this automagically in NiFi. No need to know and precreate tables. NiFi will determine the fields for you.
https://www.transportforireland.ie/transitData/Data/GTFS_Realtime.zip
GTFS Static Data Load
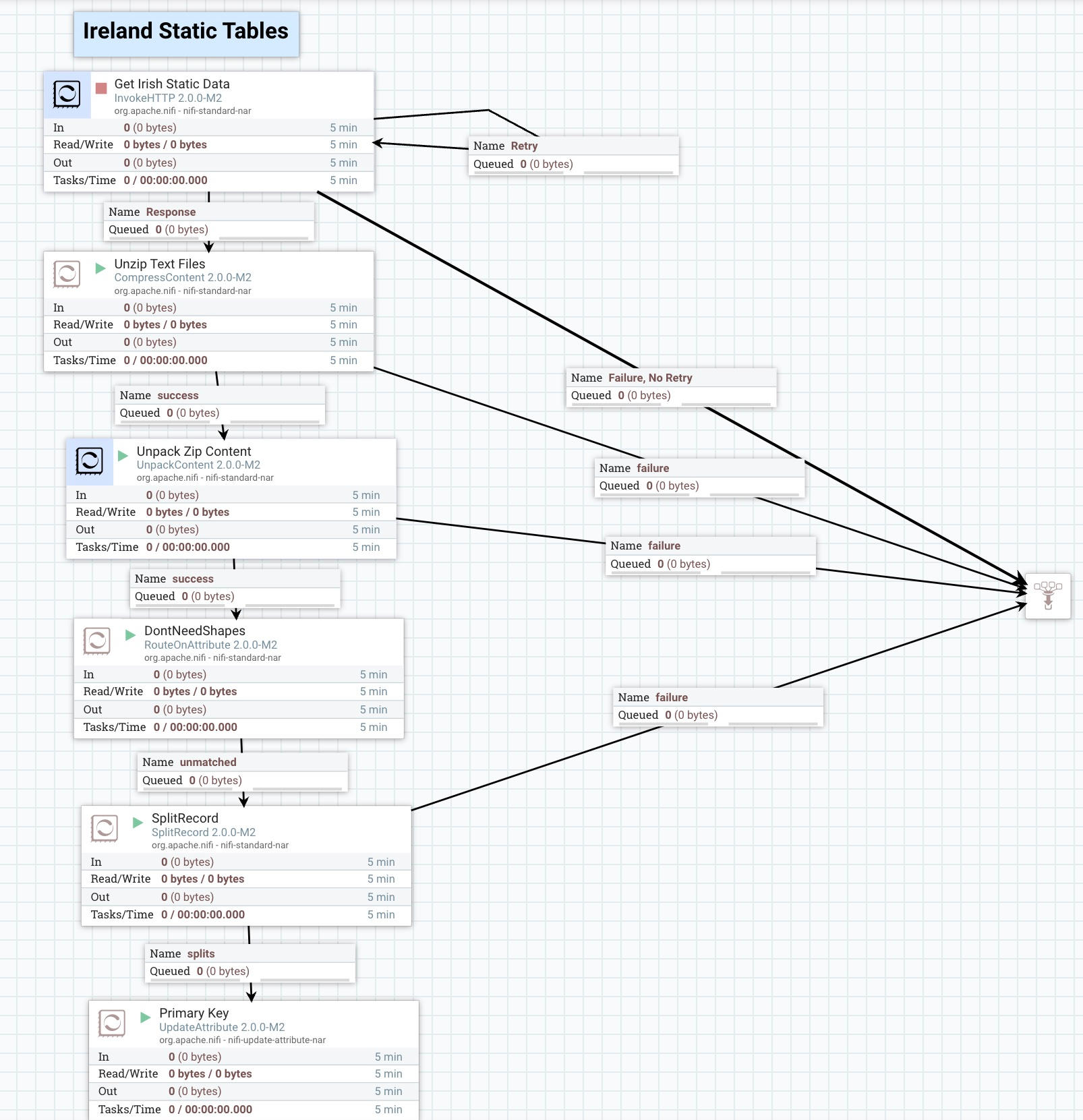
Skip shapes.txt as we aren’t loading those
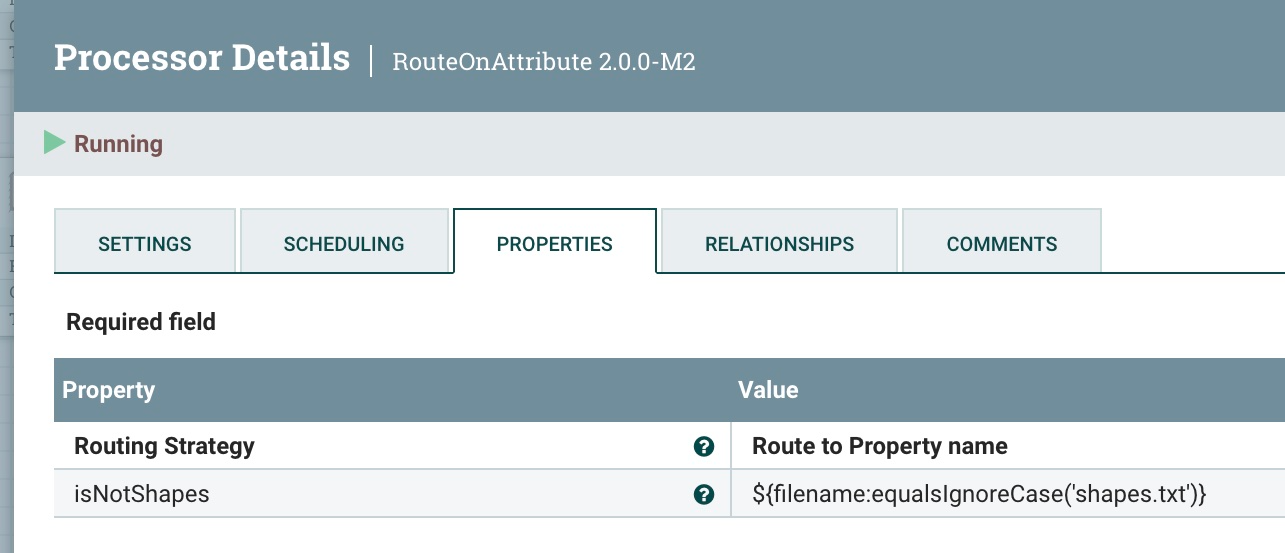
Set a Default Primary Key
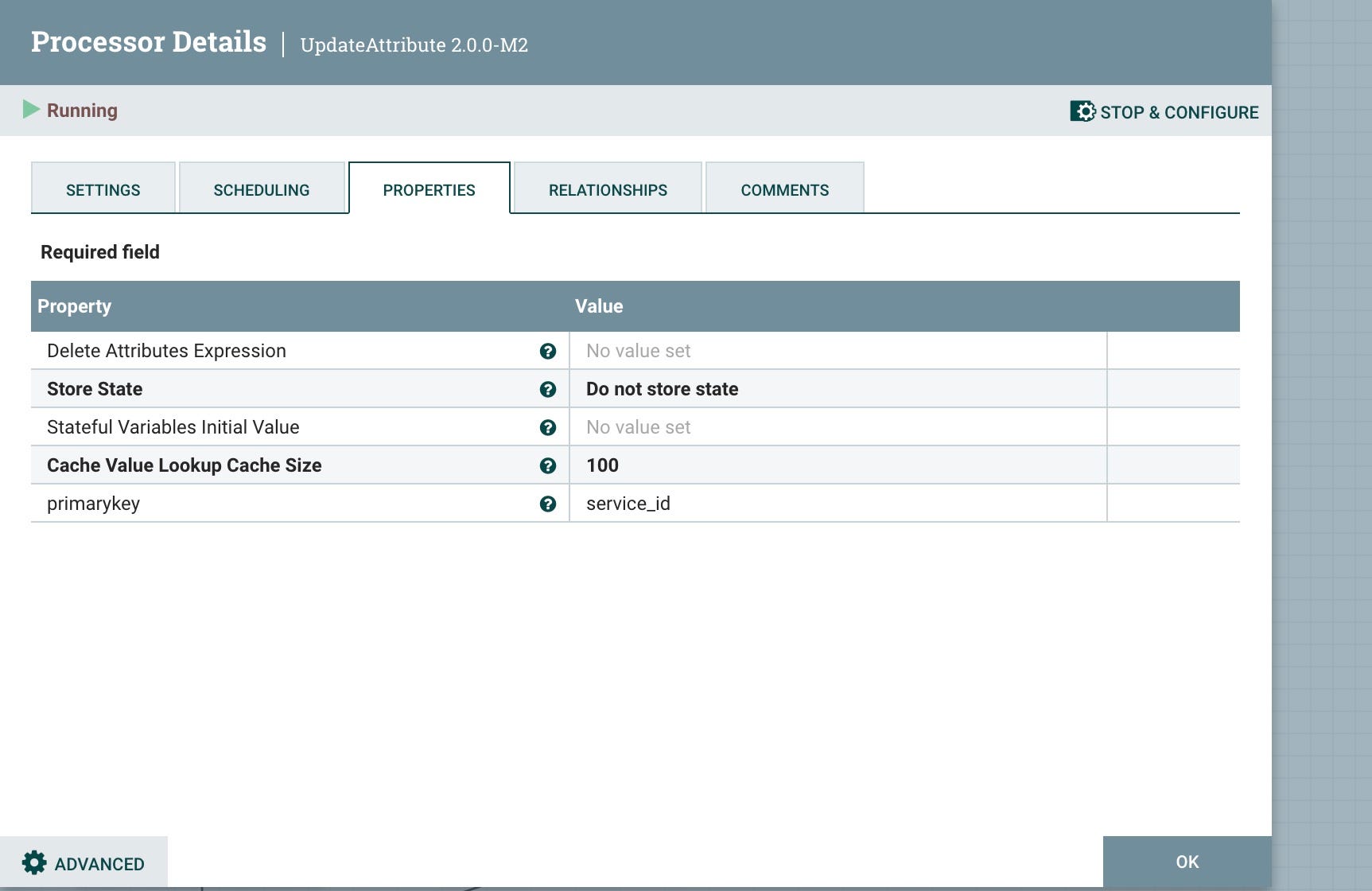
Setting All the Correct Primary Keys for all the Static Files/Tables
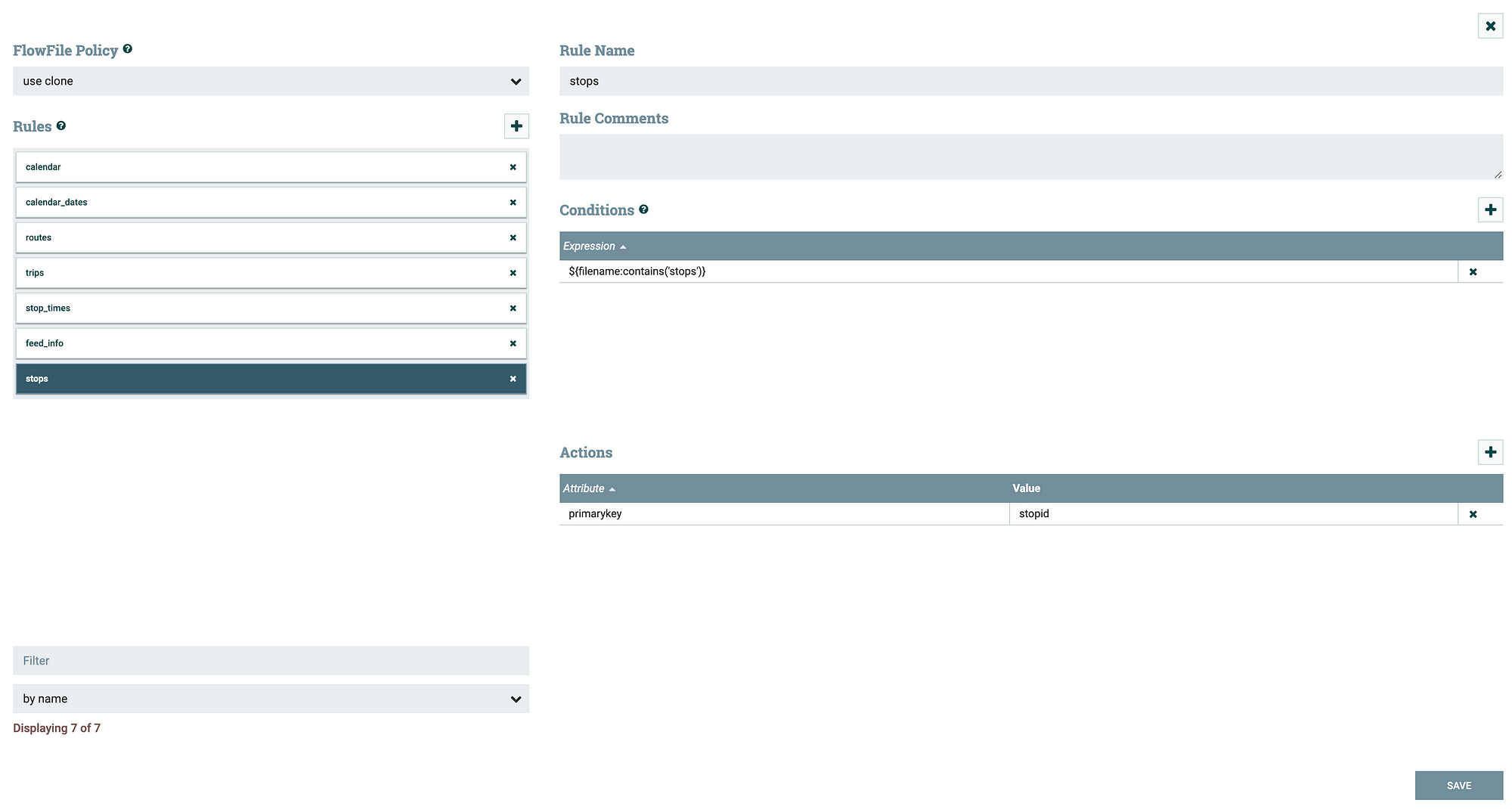
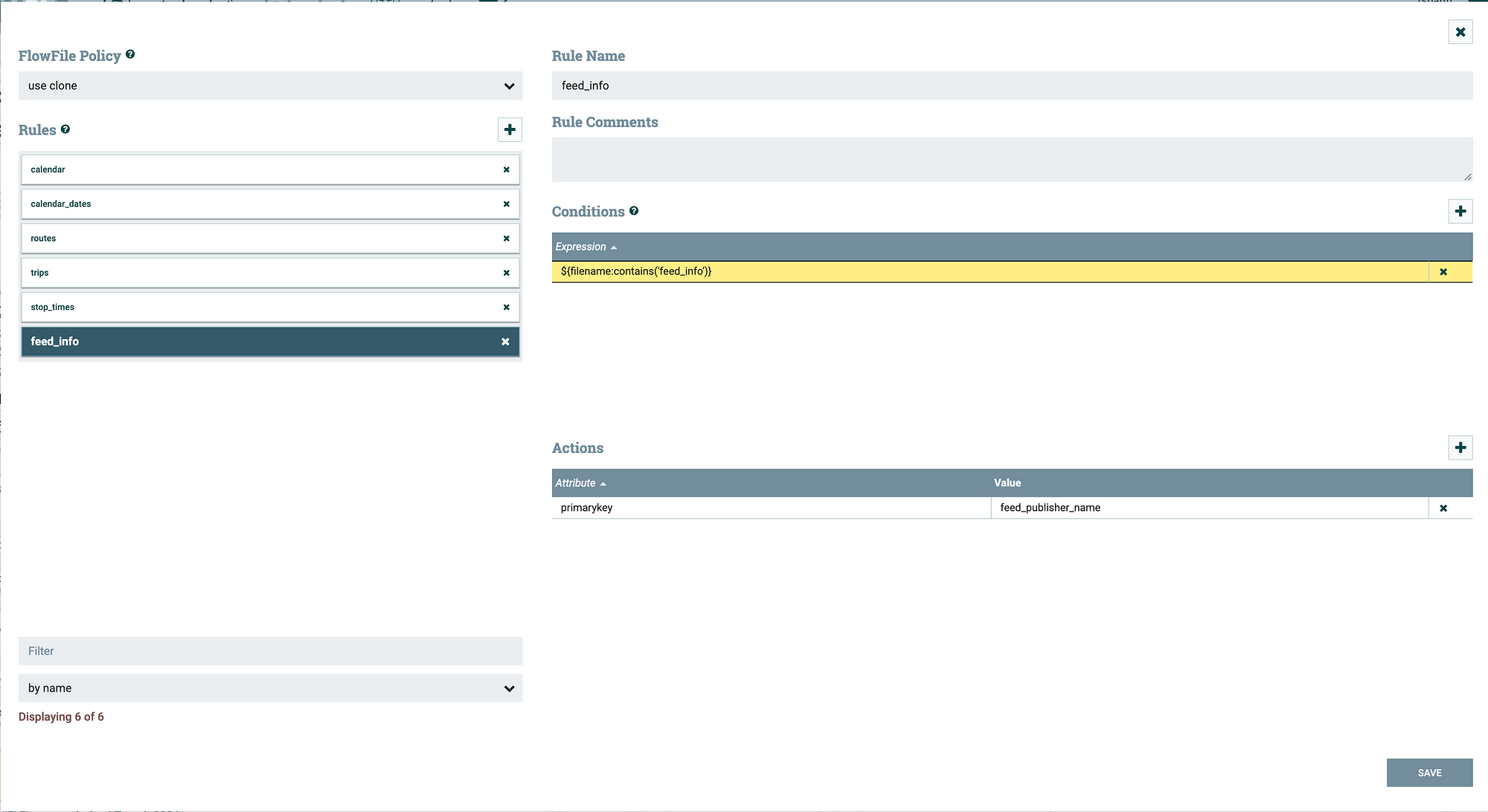

Split Up Tables into 1,000 Row Chunks to Make it Easier for Postgresql
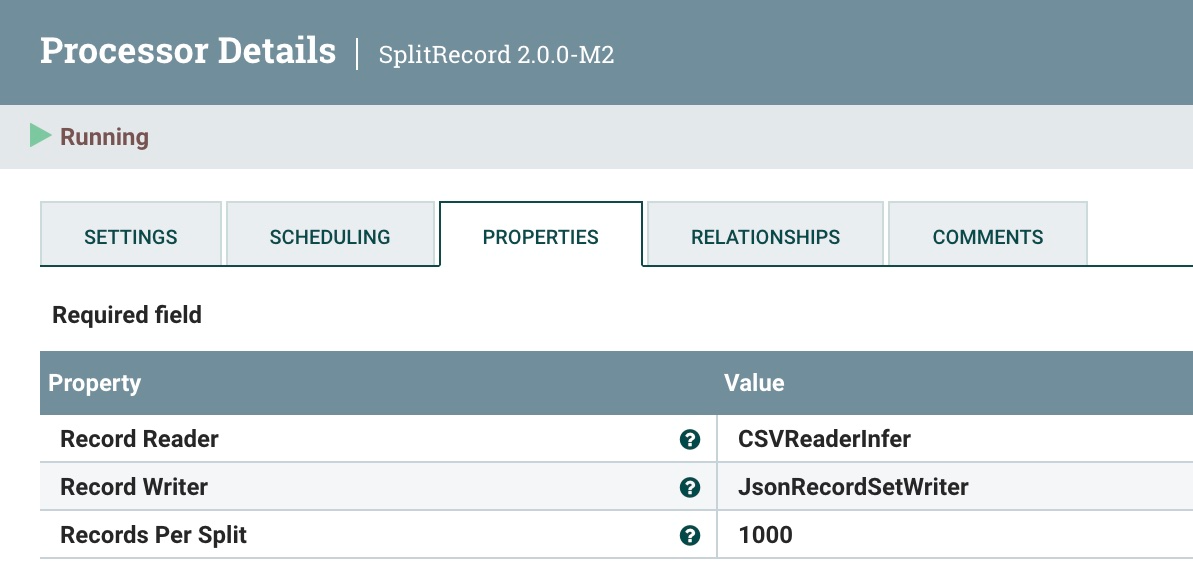
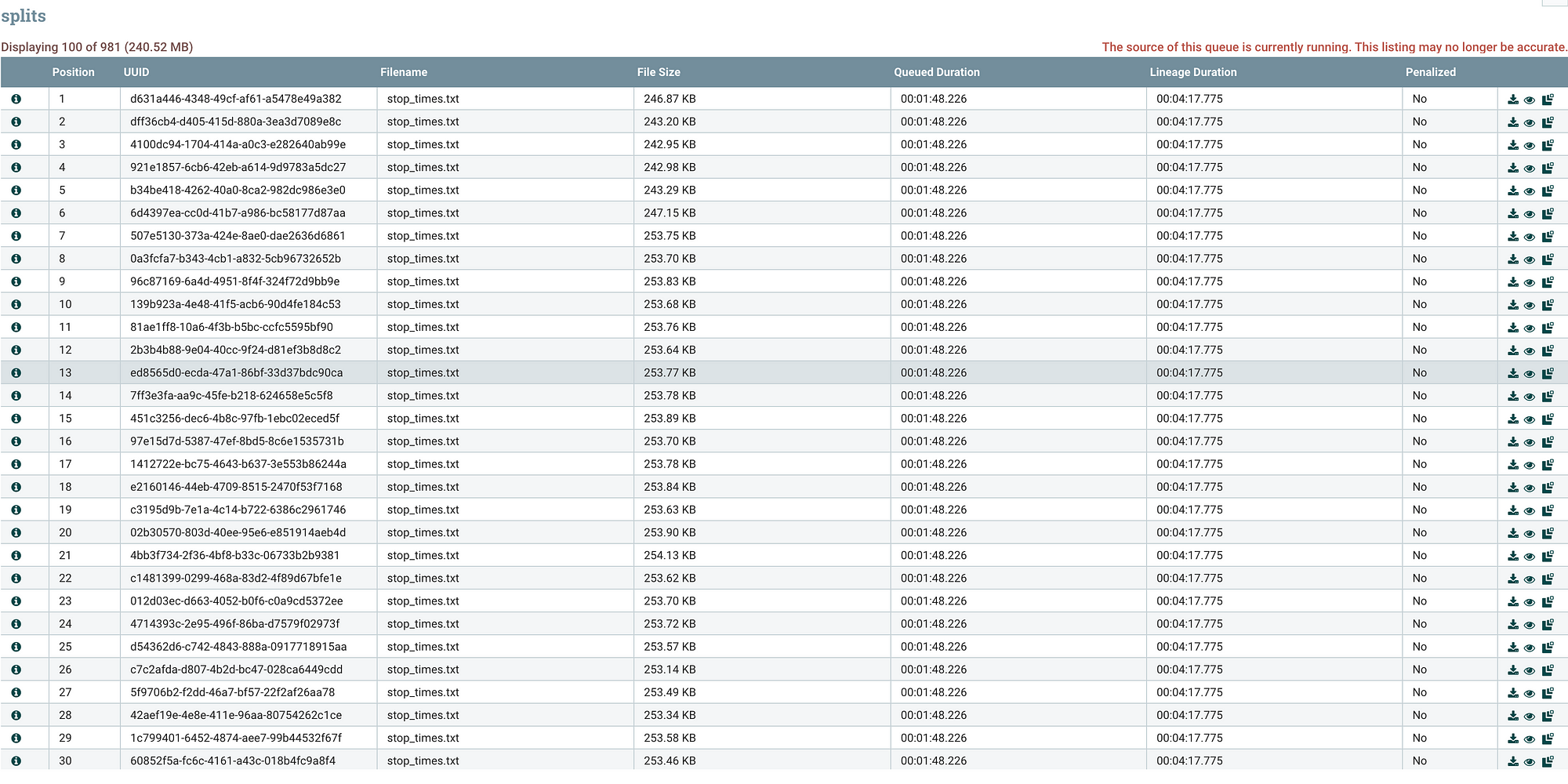
Update the SQL Automagically
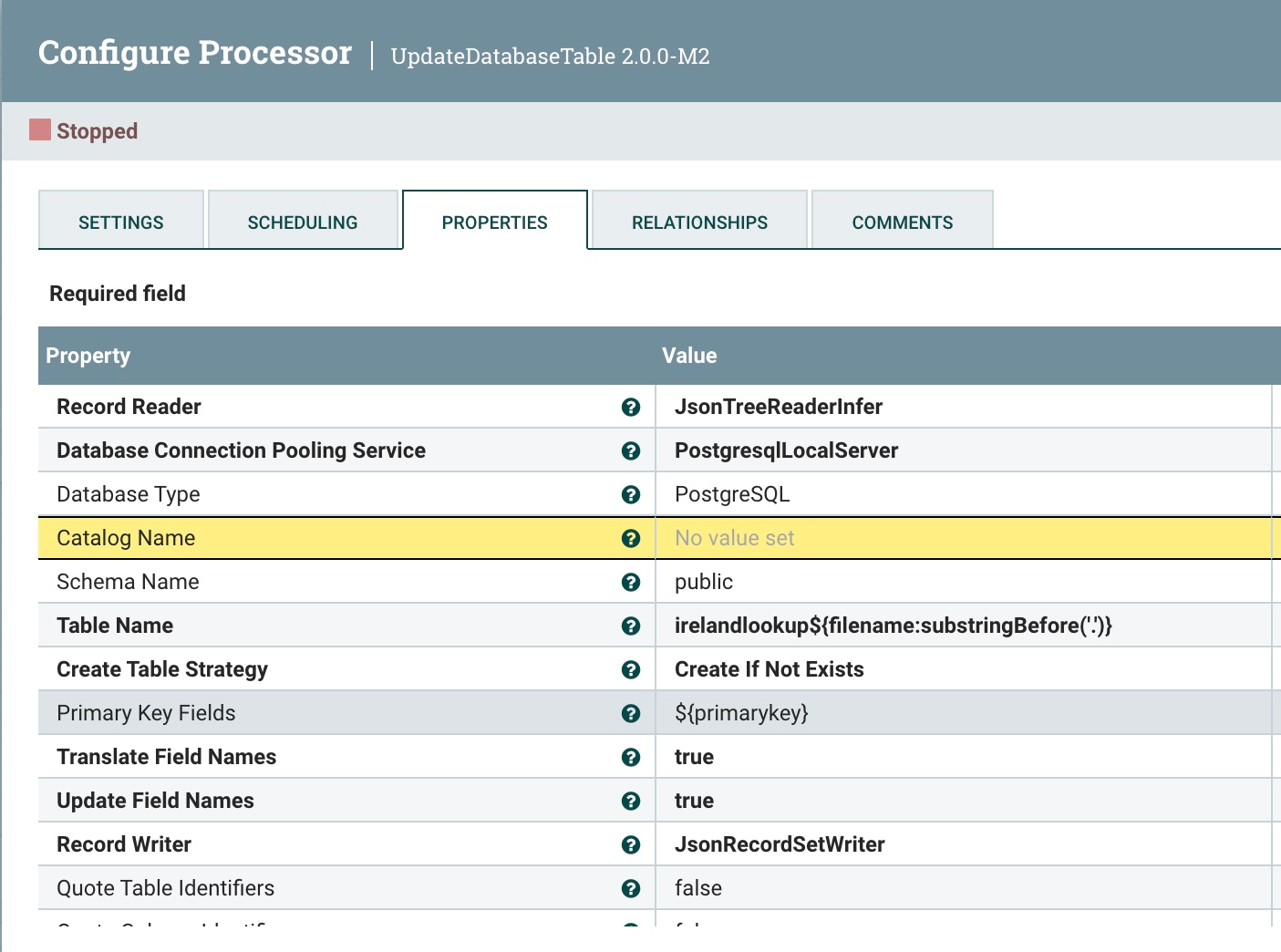
Send this SQL to the Database
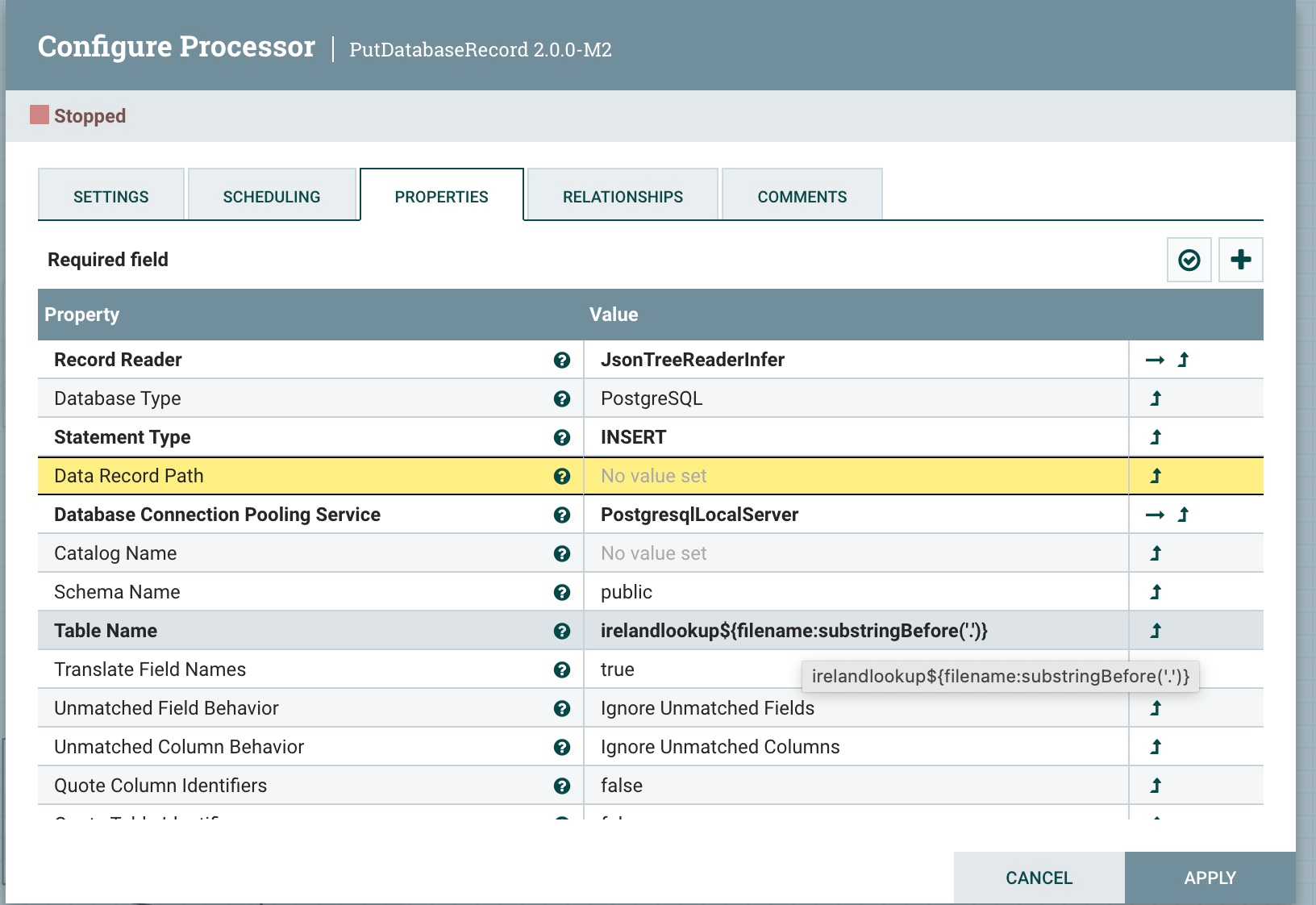
A list of Ireland Lookup Trips loaded from trips.txt
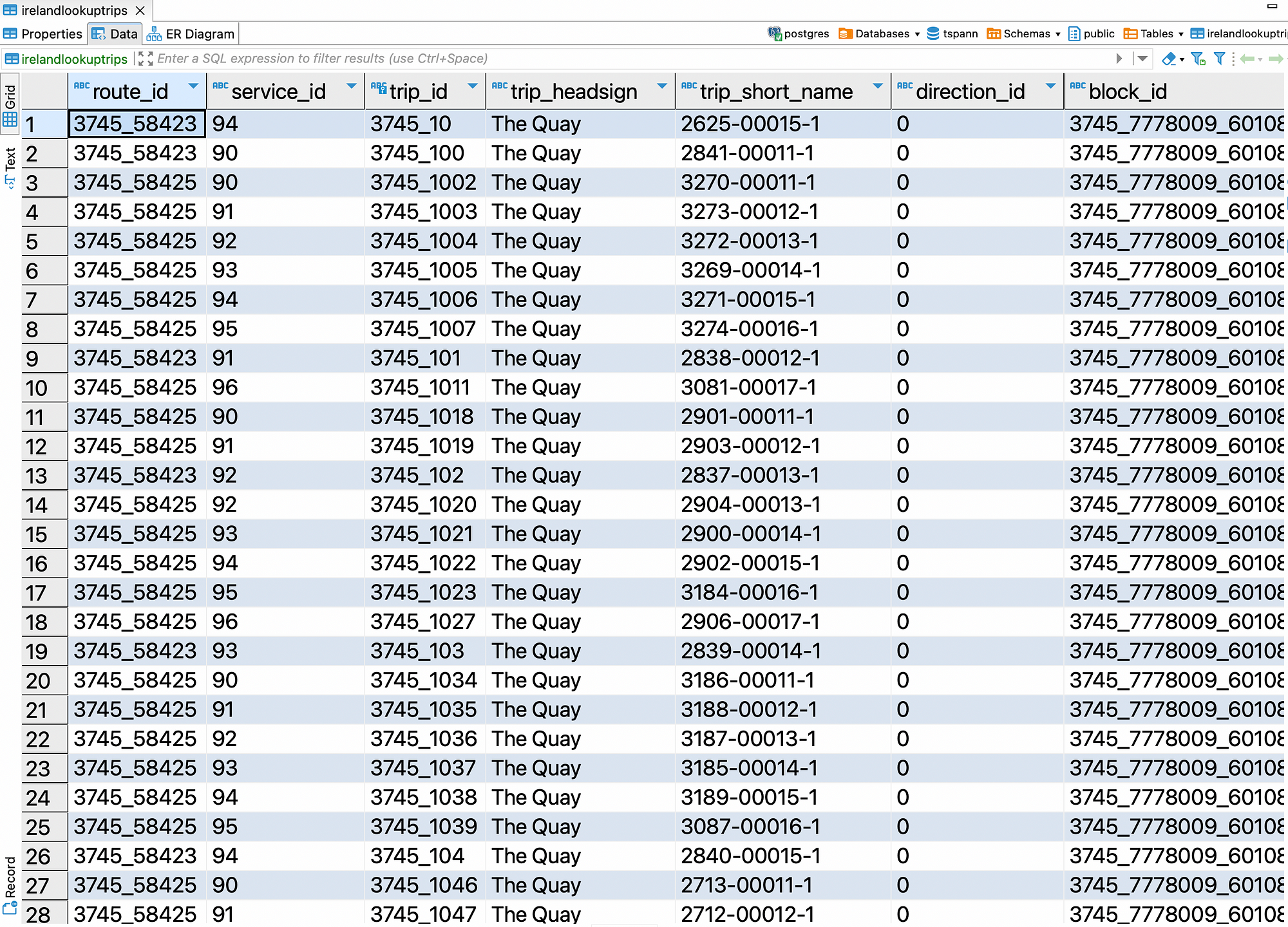
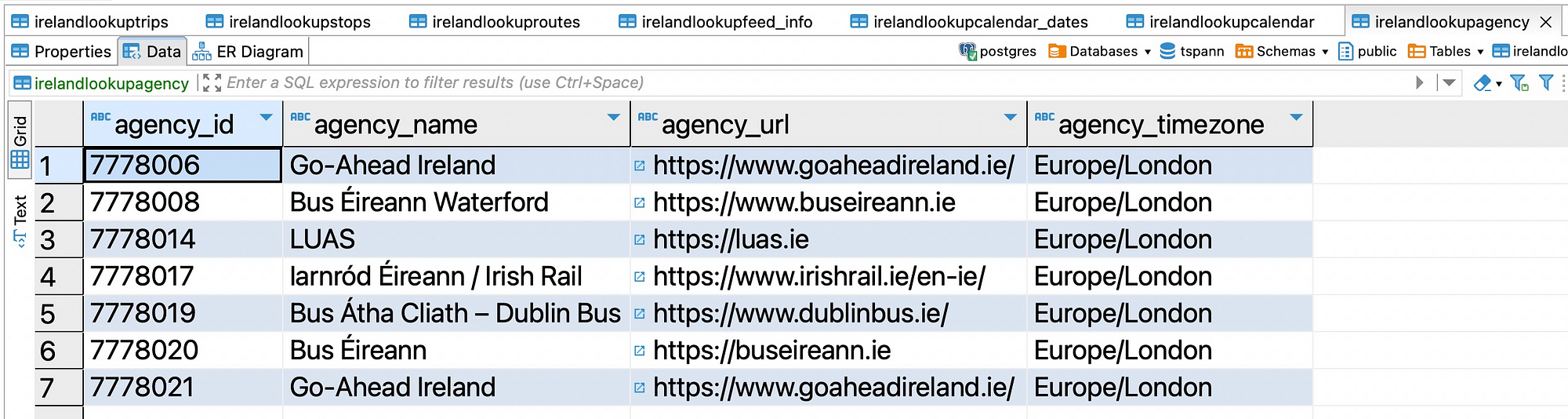
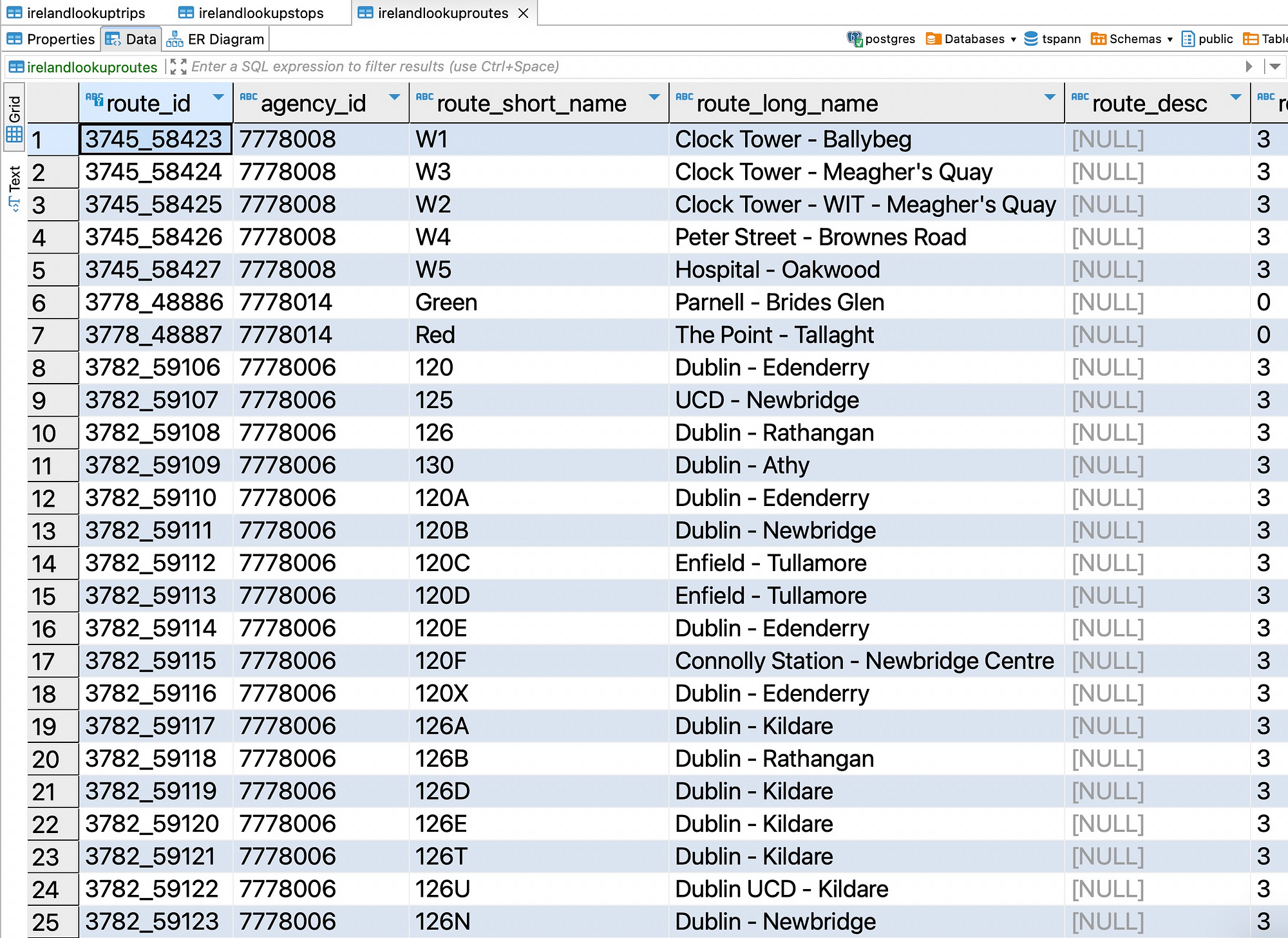
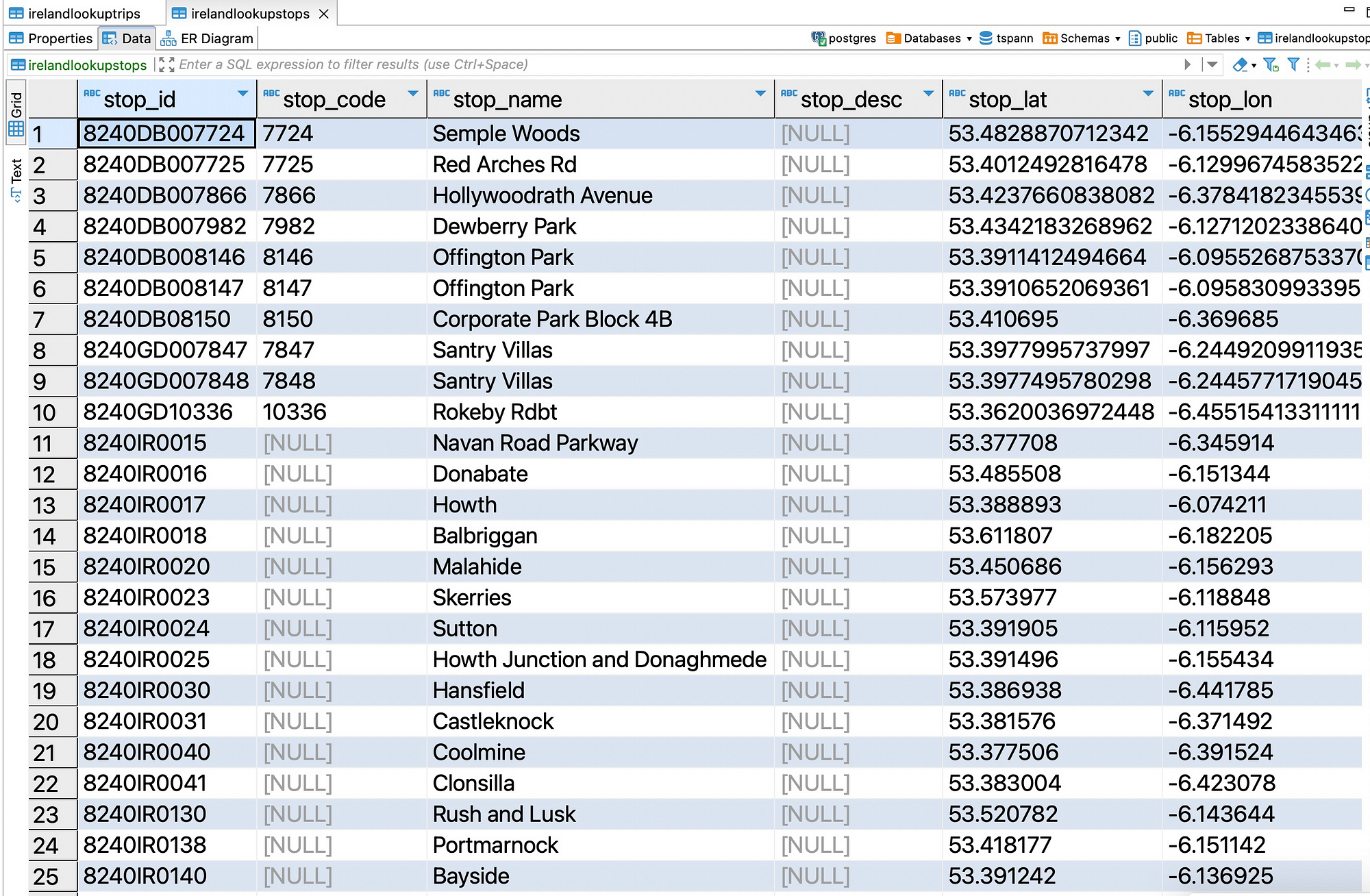
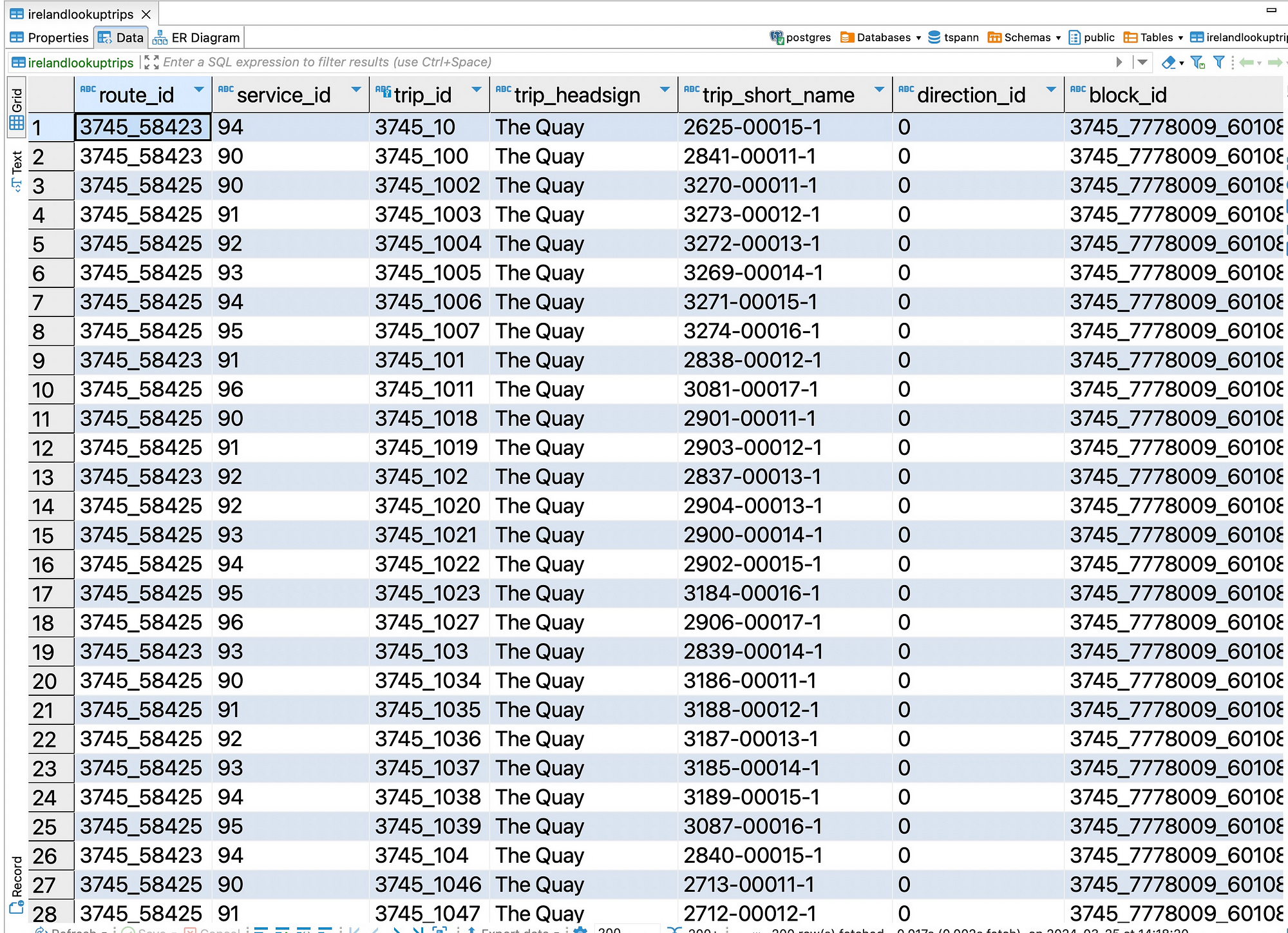
Let’s parse the real time transit information for Ireland.
GTFS Real-Time
Vehicle Positions is the primary API to get where the buses are.
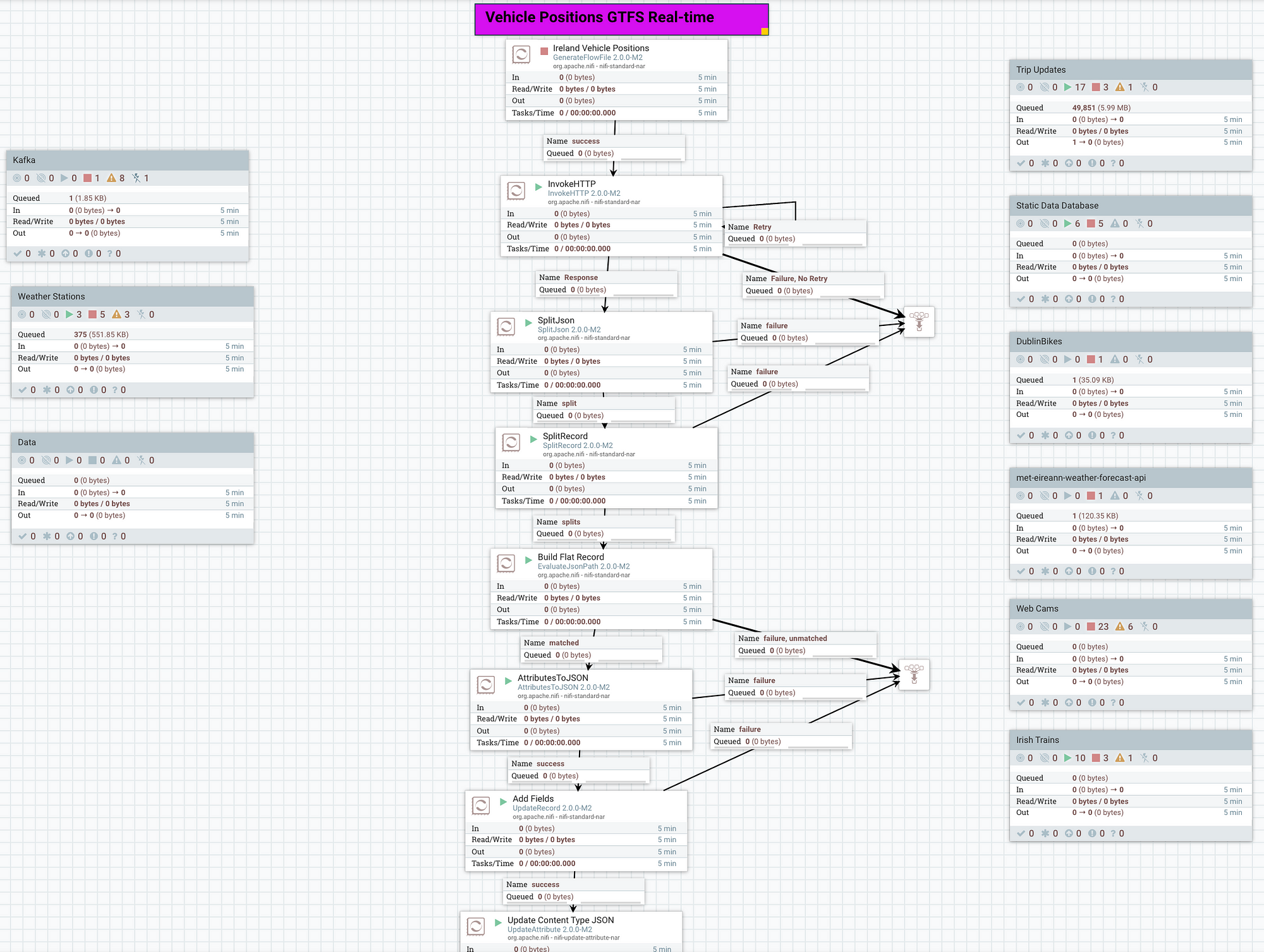
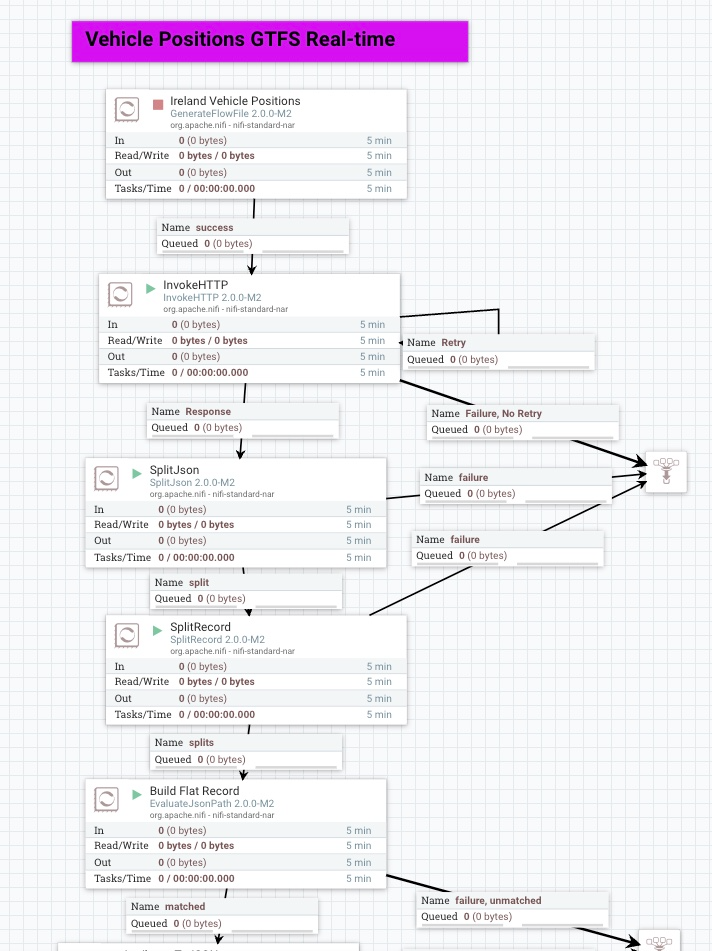
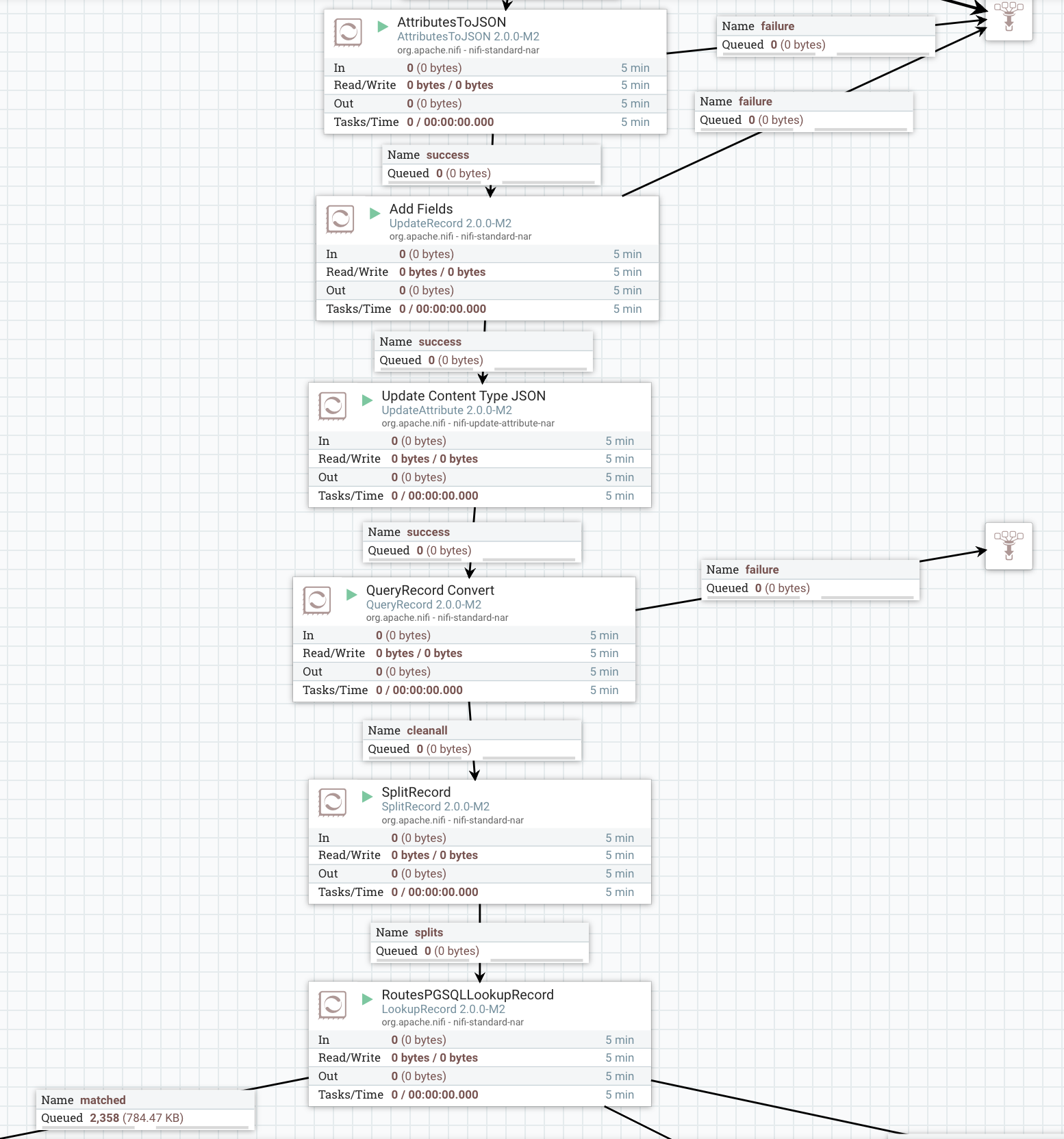
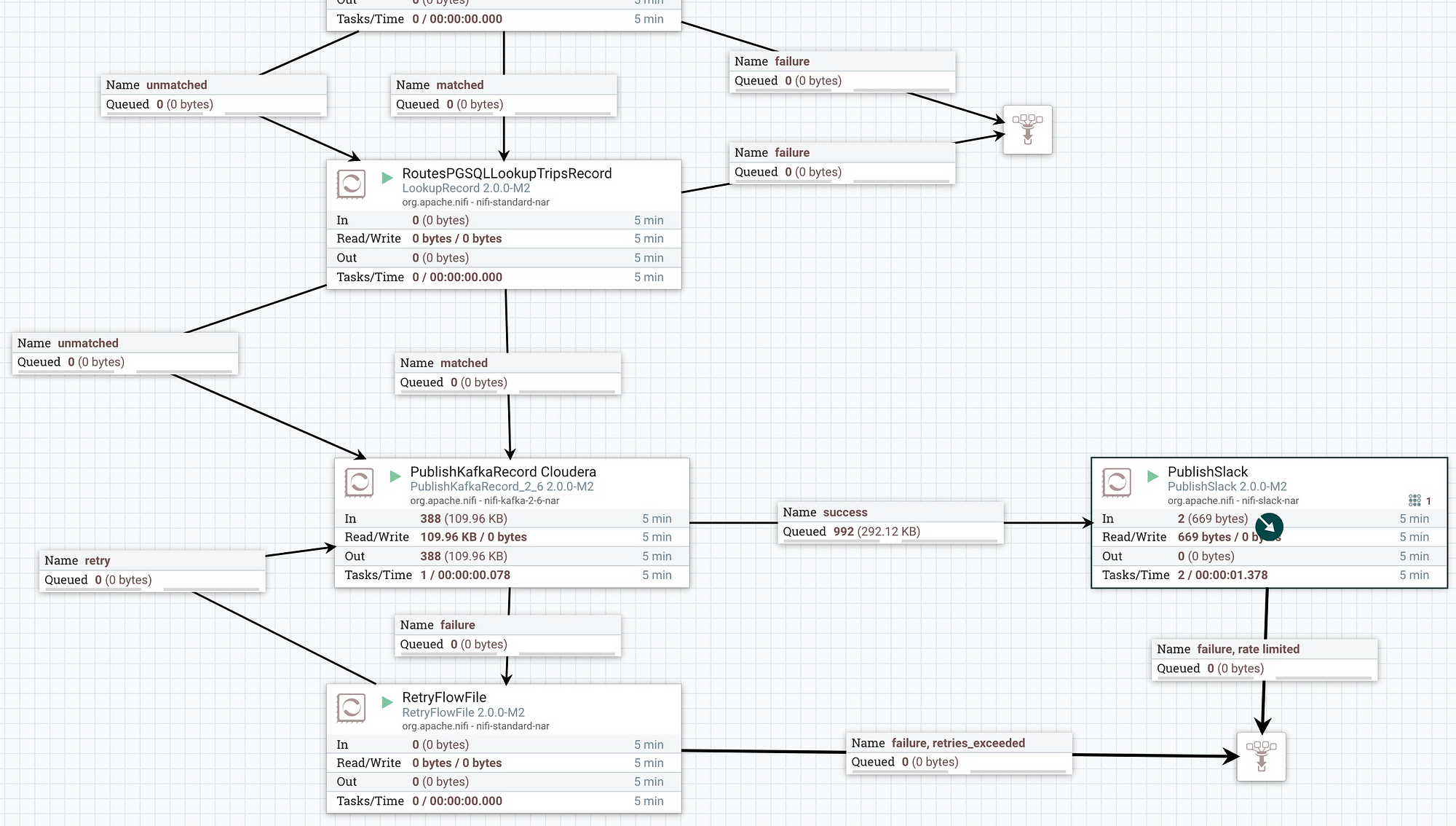
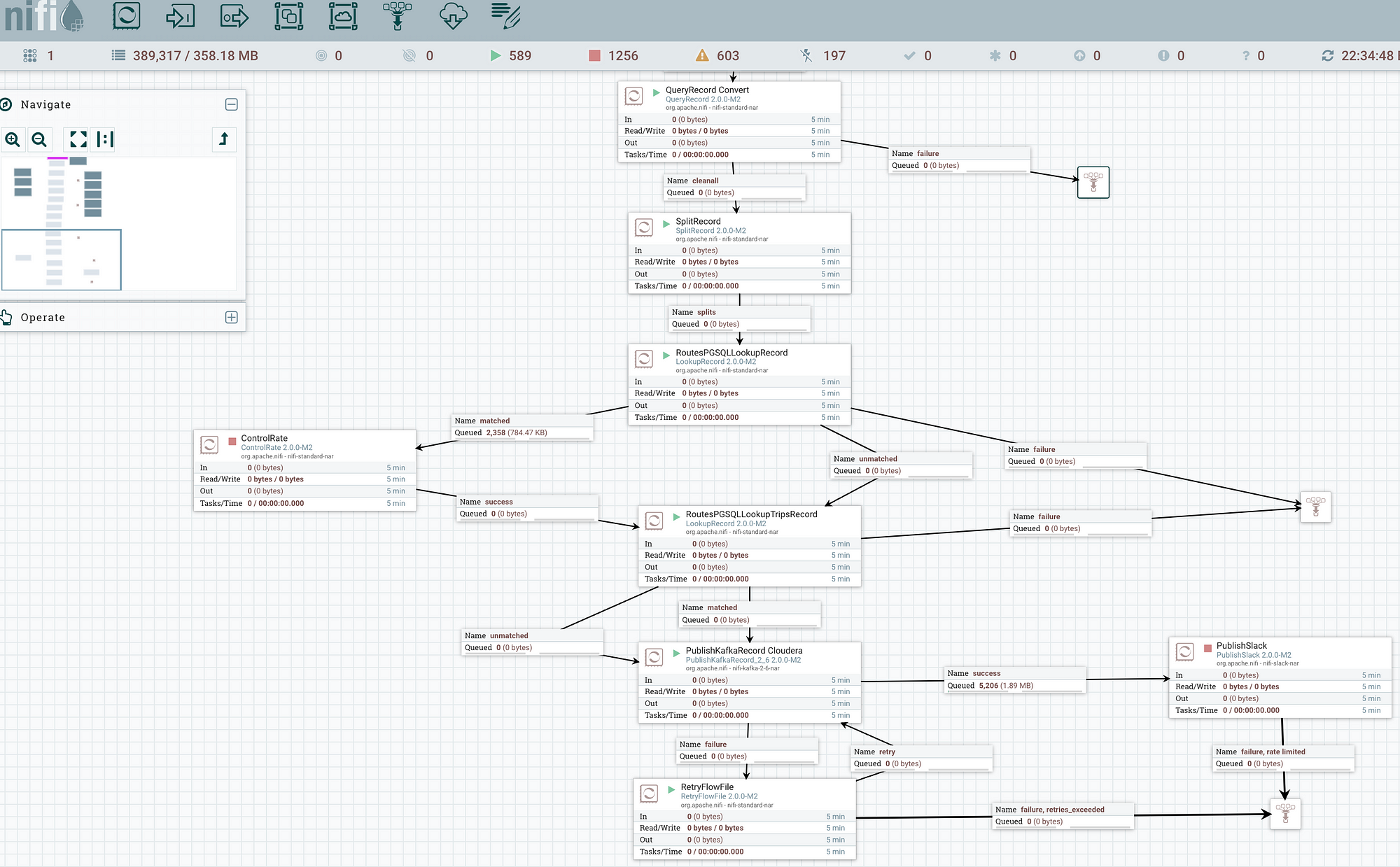
API REST TEST
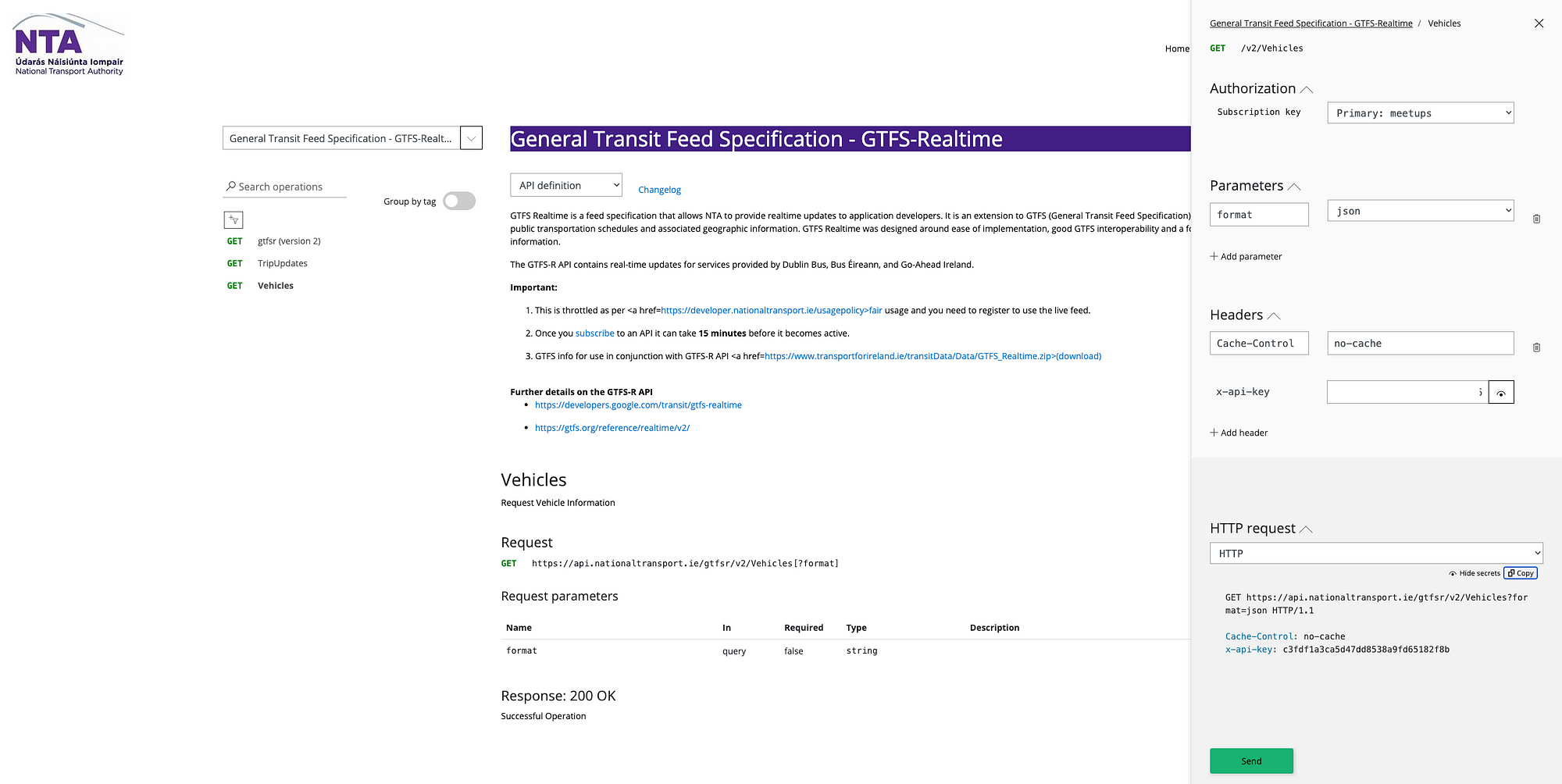
Discover APIs, learn how to use them, try them out interactively, and sign up to acquire keys.developer.nationaltransport.ie
GET https://api.nationaltransport.ie/gtfsr/v2/gtfsr?format=json HTTP/1.1
Cache-Control: no-cache
x-api-key: dddddd
As opposed to most transit systems we have seen in GTFS and GTFS-R feeds they don’t have three types, just the two. They are missing alerts.
[ Trip Updates, Vehicle Positions]
Discover APIs, learn how to use them, try them out interactively, and sign up to acquire keys.developer.nationaltransport.ie
Discover APIs, learn how to use them, try them out interactively, and sign up to acquire keys.developer.nationaltransport.ie
The GTFS-R API contains real-time updates for services provided by Dublin Bus, Bus Éireann, and Go-Ahead Ireland.
You have to sign up and subscribe to the API to use this.
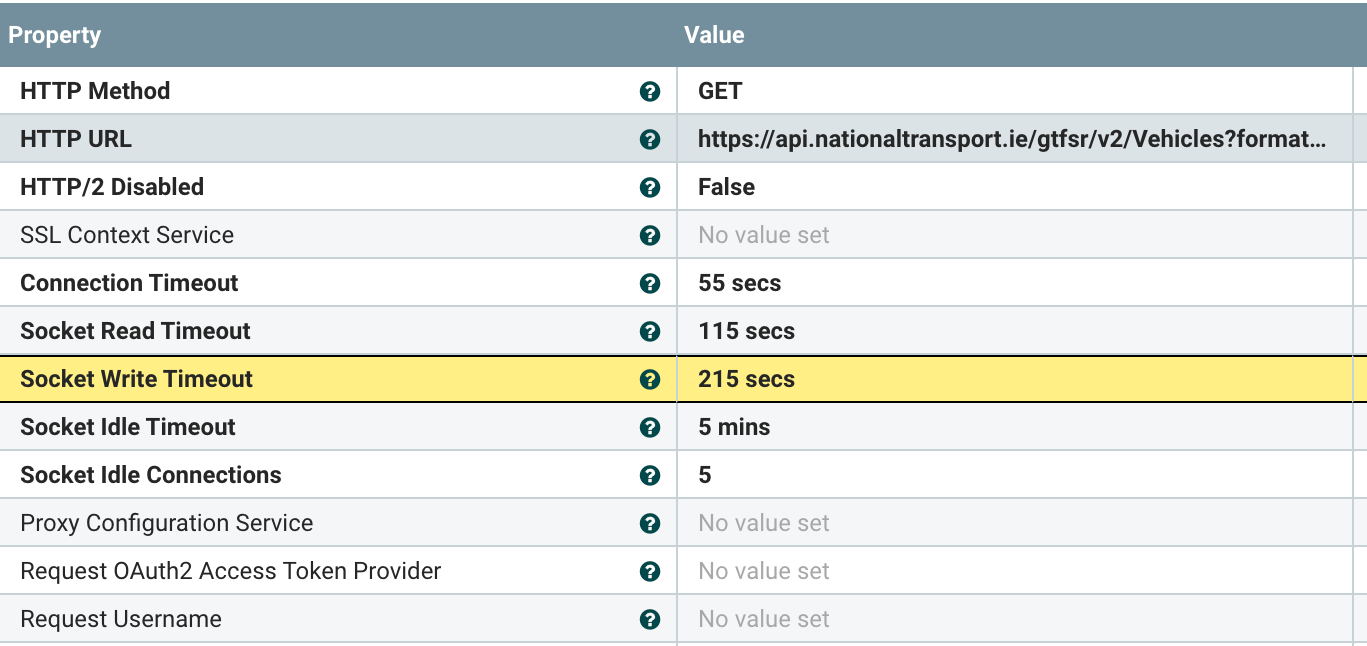
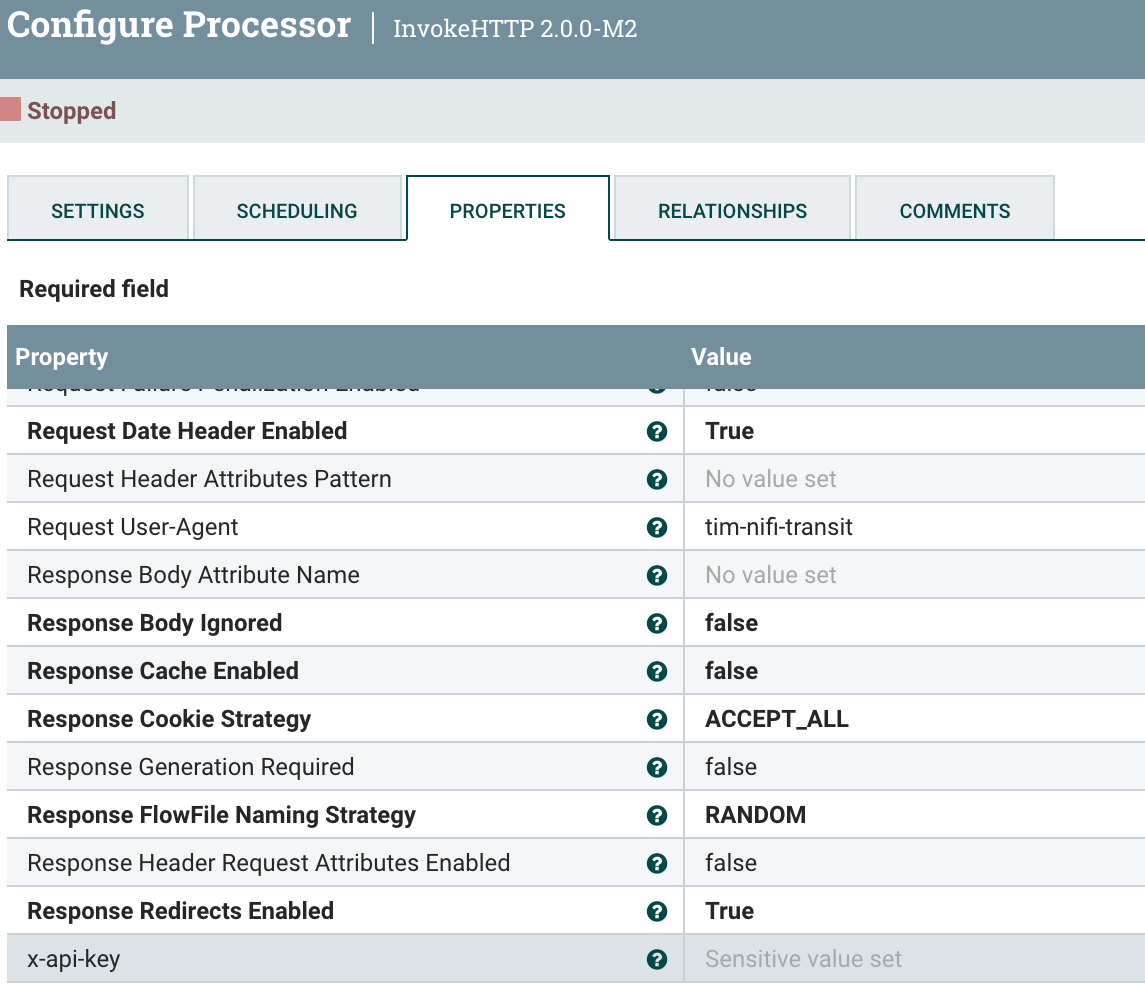
Example Vehicle Position as JSON
[ {
"recordid" : "V56",
"route_id" : "3924_62692",
"directionid" : "0",
"latitude" : "53.3537788",
"tripid" : "3924_16321",
"starttime" : "22:50:00",
"vehicleid" : "274",
"startdate" : "20240322",
"uuid" : "8a50c084-0aea-496e-b4c3-dbed373e812e",
"longitude" : "-6.40118694",
"timestamp" : "1711150967",
"ts" : "1711167213555"
} ]
Vehicle Position Slack Message
Irish Transit Tracking
Direction ${directionid}
Request ${invokehttp.request.url} ${invokehttp.status.message} ${invokehttp.tx.id}
Lat/Long ${latitude}/${longitude}
Vehicle ${vehicleid}
Route ${route_id}
Scheduled? ${scheduled}
Start Date/Time/TS ${startdate} / ${starttime} / ${timestamp}
IDs ${uuid} ${recordid} TripID ${tripid}
Scheduled: ${scheduled}
Trip Updates
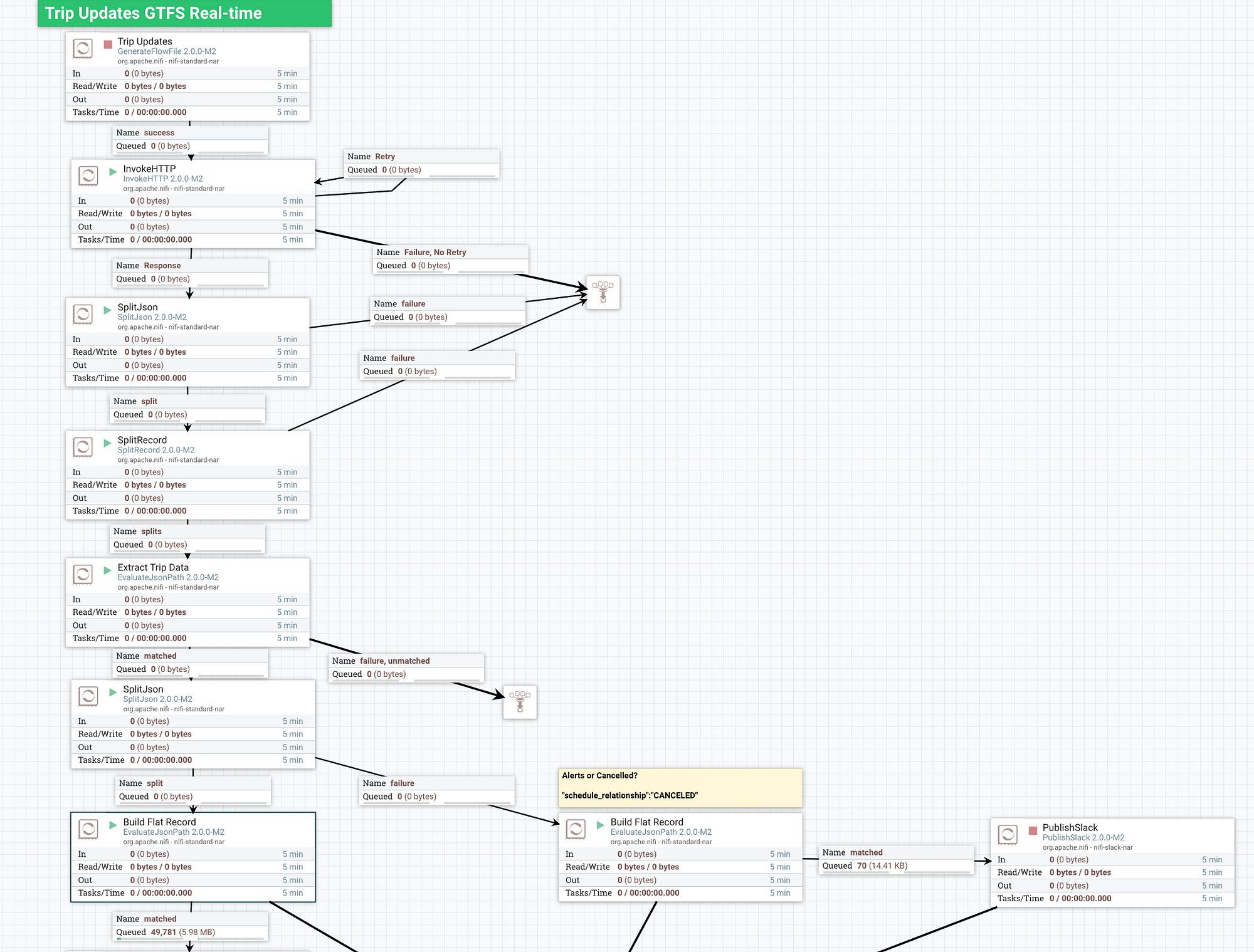
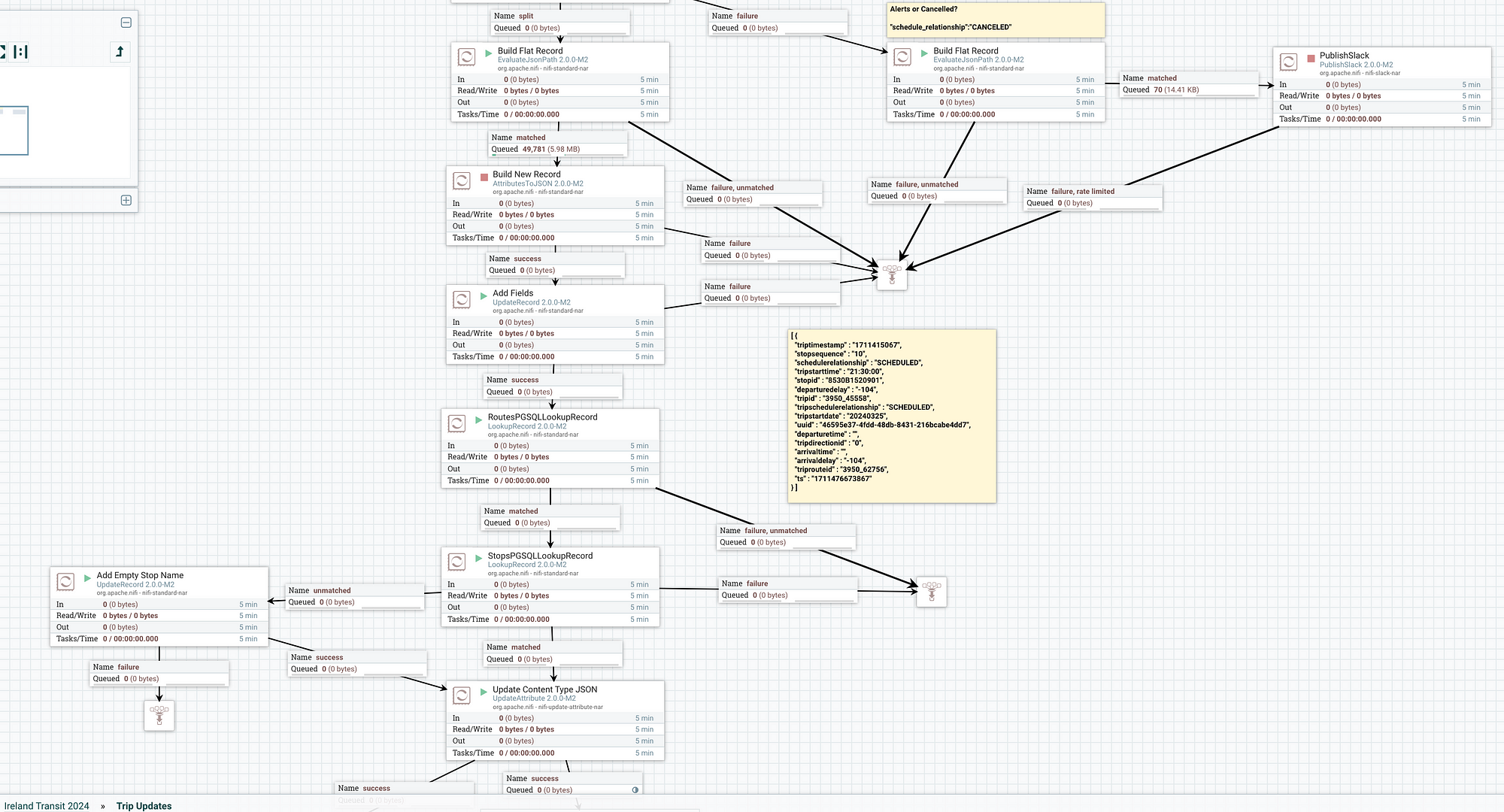
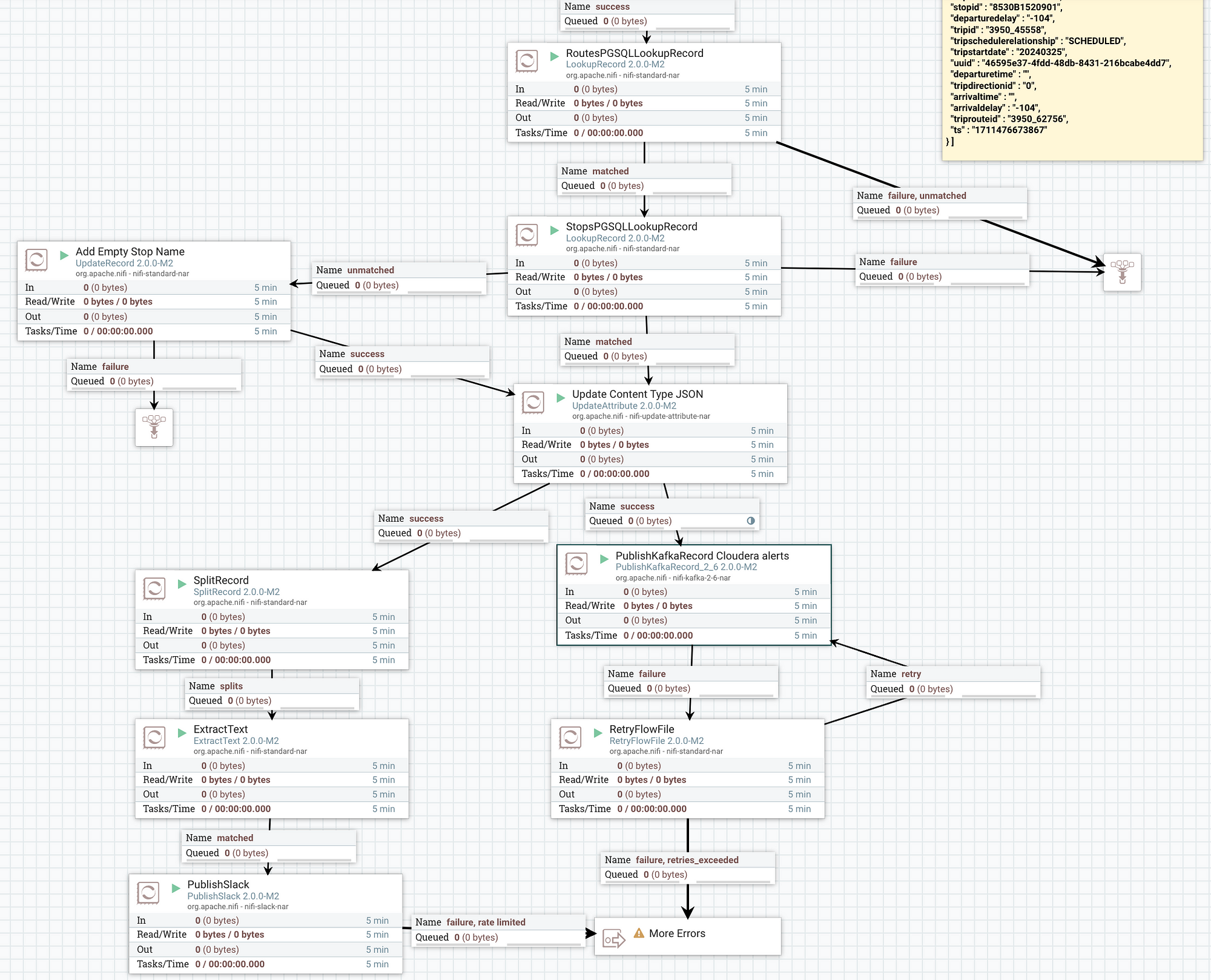
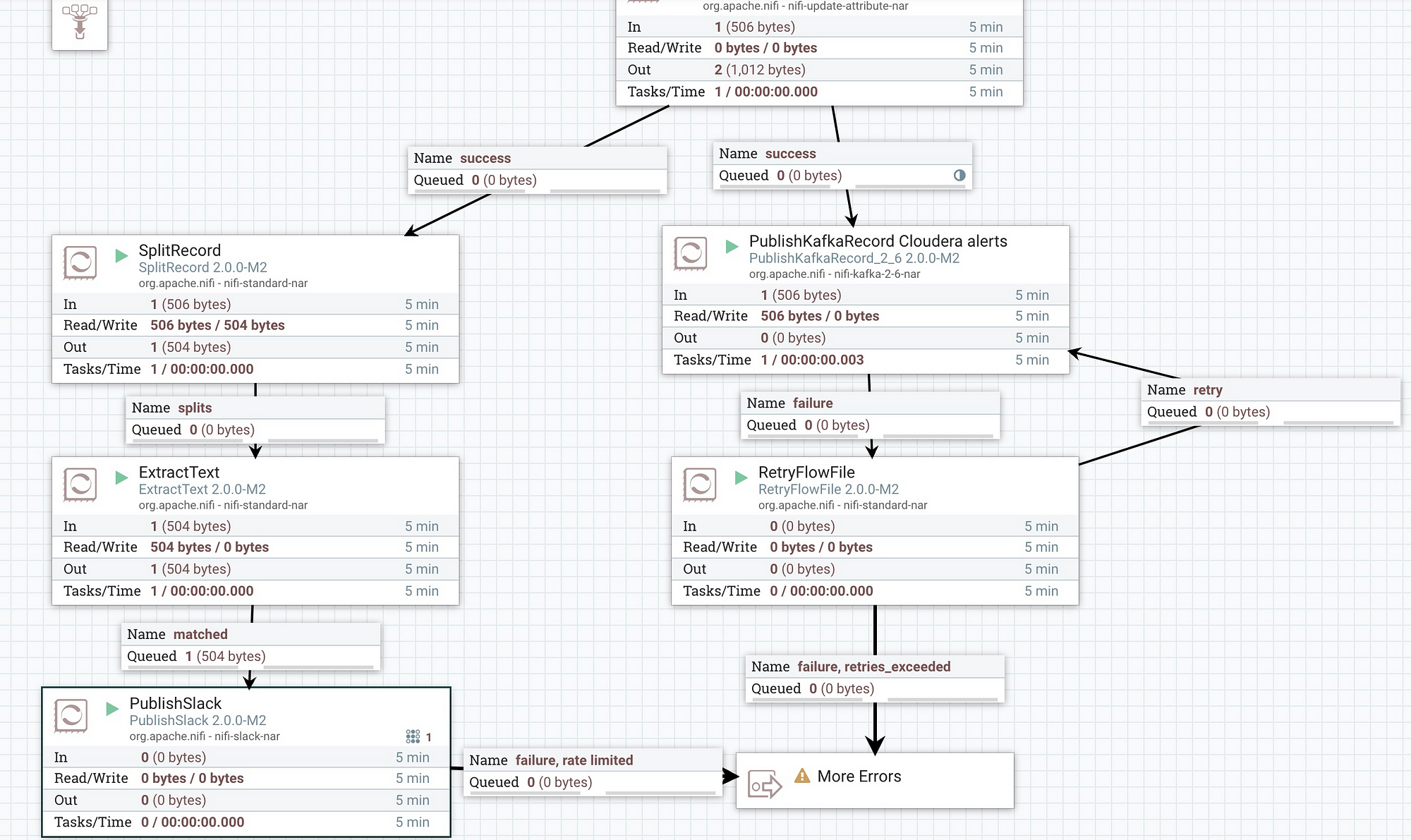
Example Trip Update as JSON
{
"triptimestamp" : "1711415067",
"stopsequence" : "10",
"schedulerelationship" : "SCHEDULED",
"tripstarttime" : "21:30:00",
"stopid" : "8530B1520901",
"departuredelay" : "-104",
"tripid" : "3950_45558",
"tripschedulerelationship" : "SCHEDULED",
"tripstartdate" : "20240325",
"uuid" : "46595e37-4fdd-48db-8431-216bcabe4dd7",
"departuretime" : "",
"tripdirectionid" : "0",
"arrivaltime" : "",
"arrivaldelay" : "-104",
"triprouteid" : "3950_62756",
"ts" : "1711476673867",
"route_long_name" : "Dublin - Airport - Cavan - Donegal",
"stop_name" : "Topaz Belleek"
}
Trip Update Slack Message
Irish Transit Tracking Trip Updates
Request ${invokehttp.request.url} ${invokehttp.status.message} ${invokehttp.tx.id}
IDs ${uuid}
Arrival Delay / Time: ${arrivaldelay} / ${arrivaltime}
Departure Delay / Time: ${departuredelay} / ${departuretime}
Schedule: ${schedulerelationship} ${tripschedulerelationship}
Stop ID/Sequence: ${stopid} / ${stopsequence}
Trip Direction: ${tripdirectionid} ${tripid}
Trip Route: ${triprouteid}
Trip Start Date / Time / TS: ${tripstartdate} / ${tripstarttime} / ${triptimestamp}
Create Table in Flink
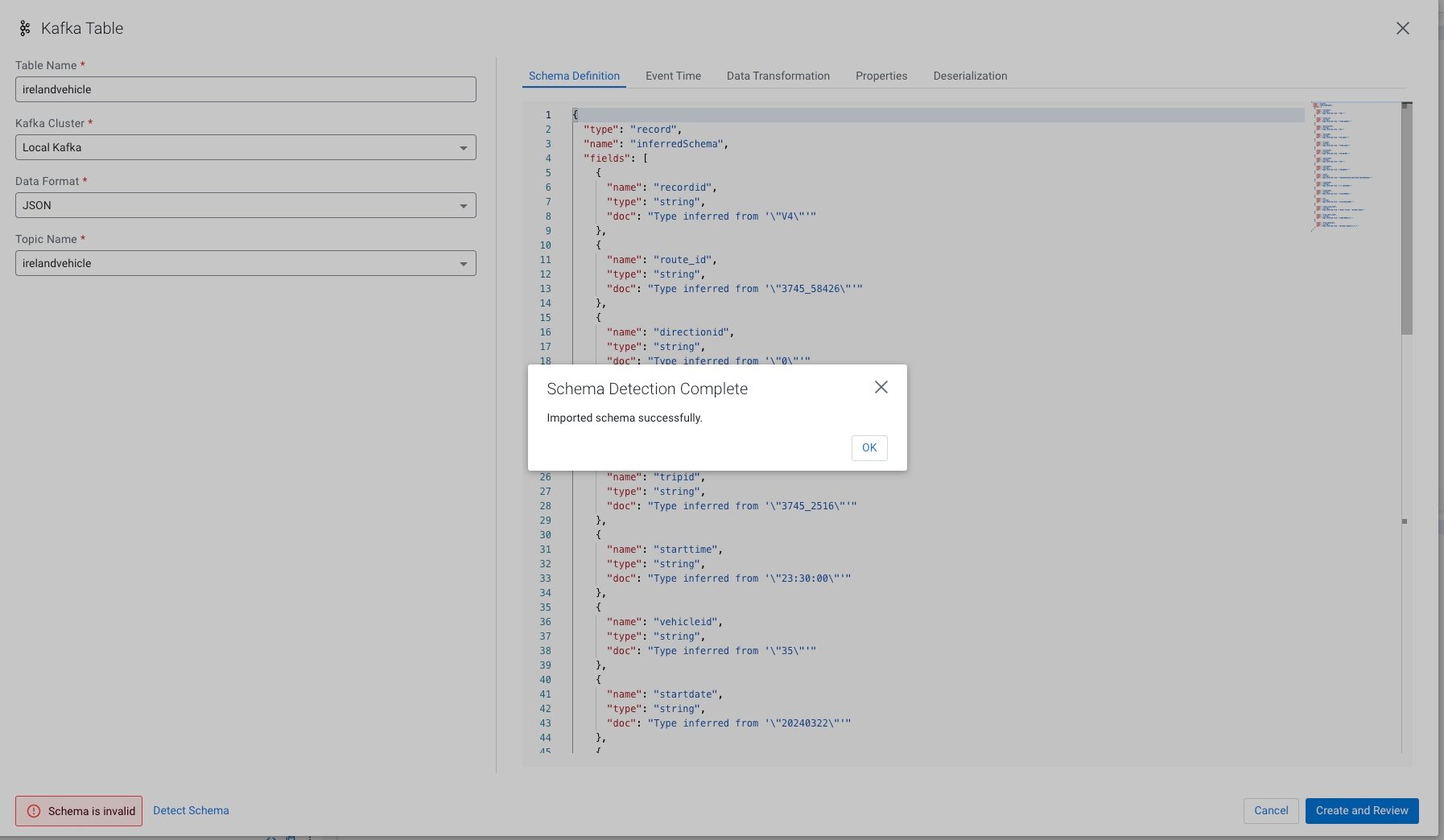
Query Kafka Topic — Flink SQL Table in SSB
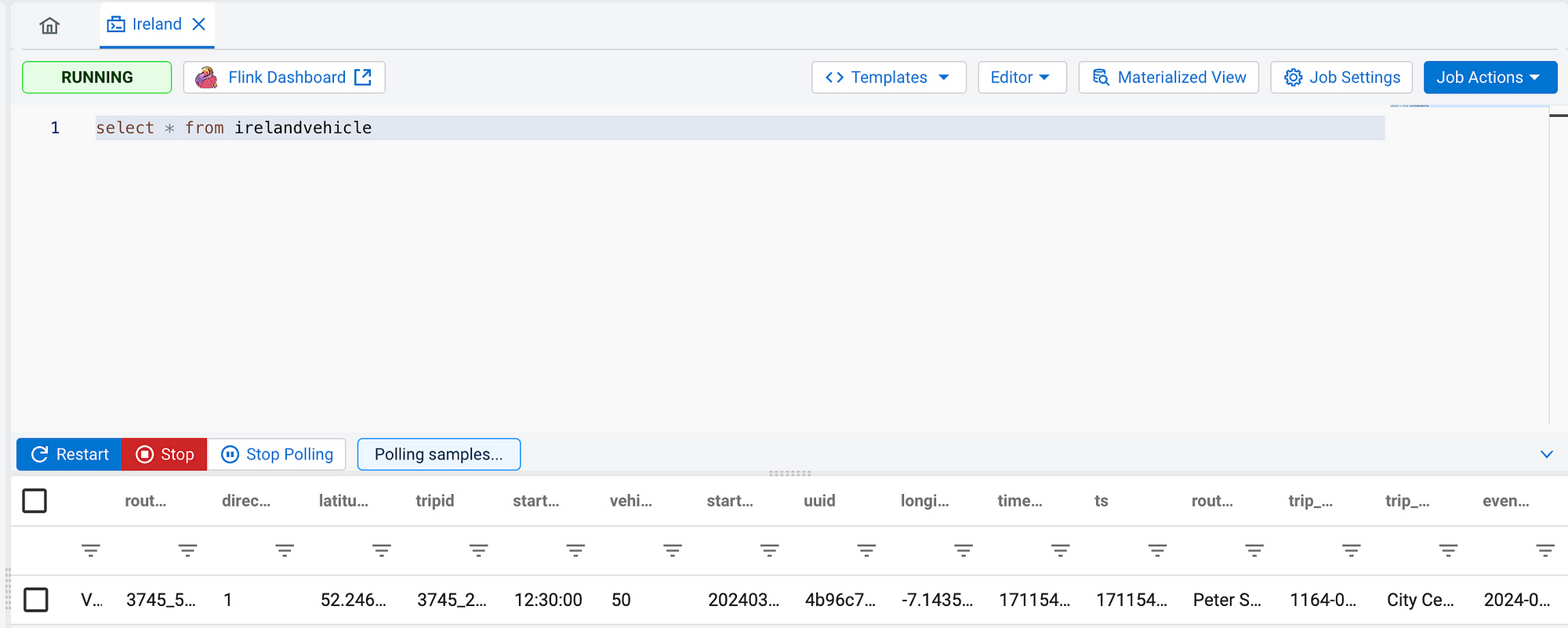
You need to access the Downloads Page of Cloudera Stream Processing (CSP) to download the Community Edition version of…docs.cloudera.com
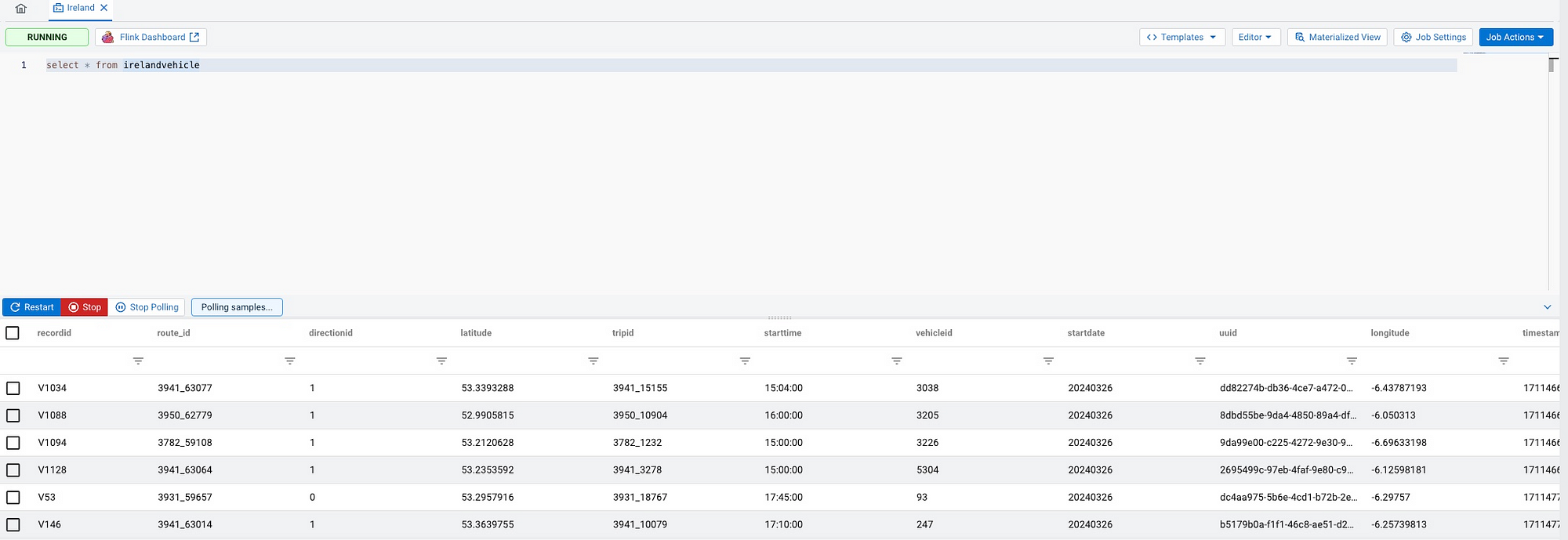
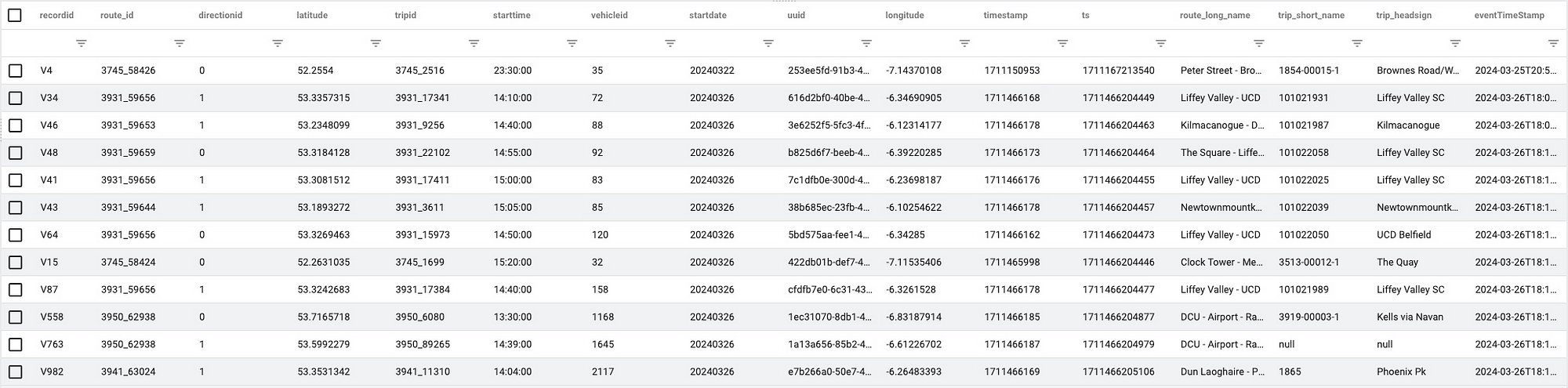
Send Messages
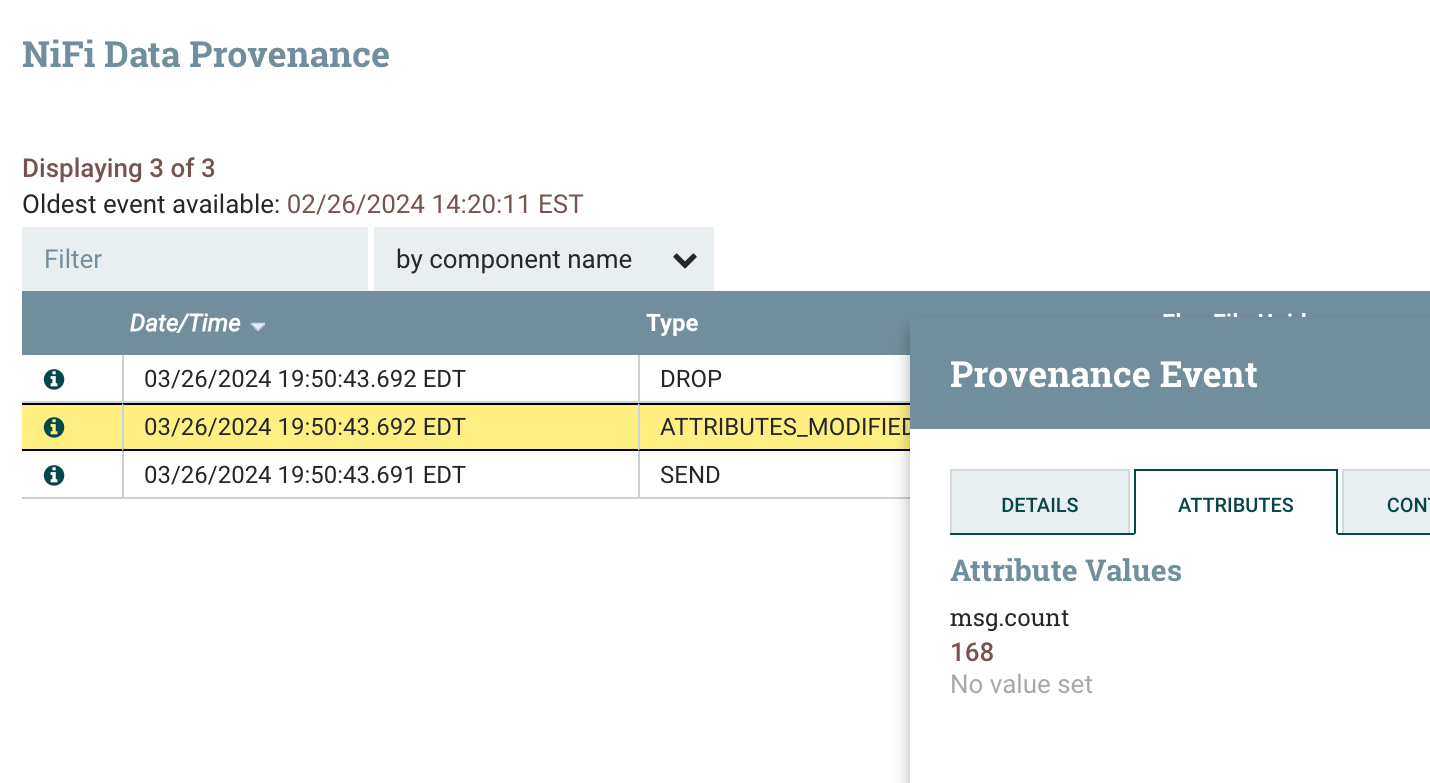
Lookups From Postgresql Table

Finally Send Messages to Slack
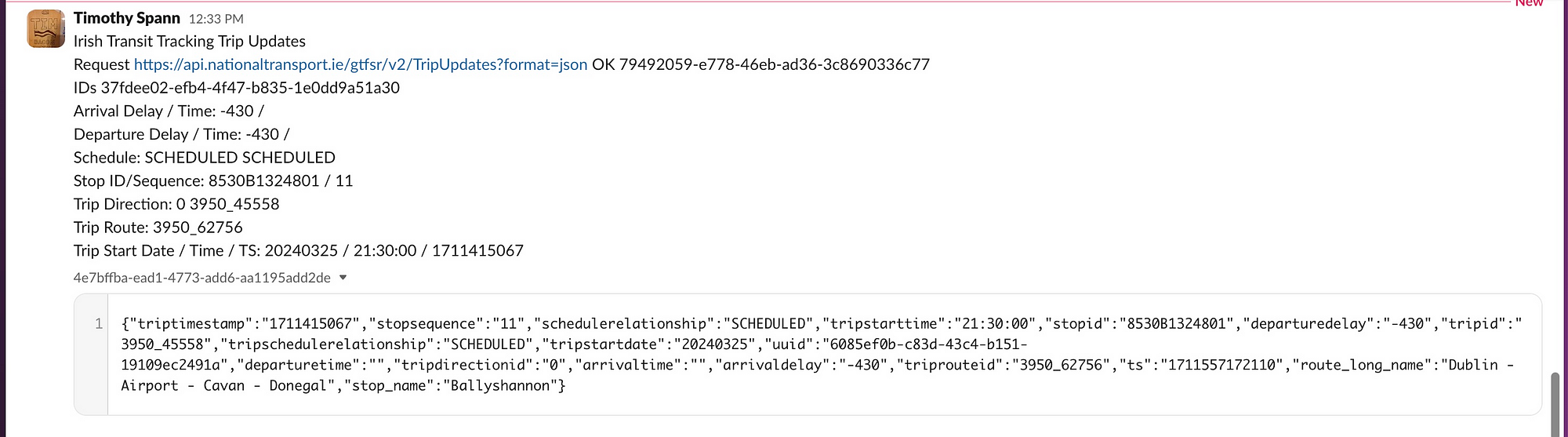
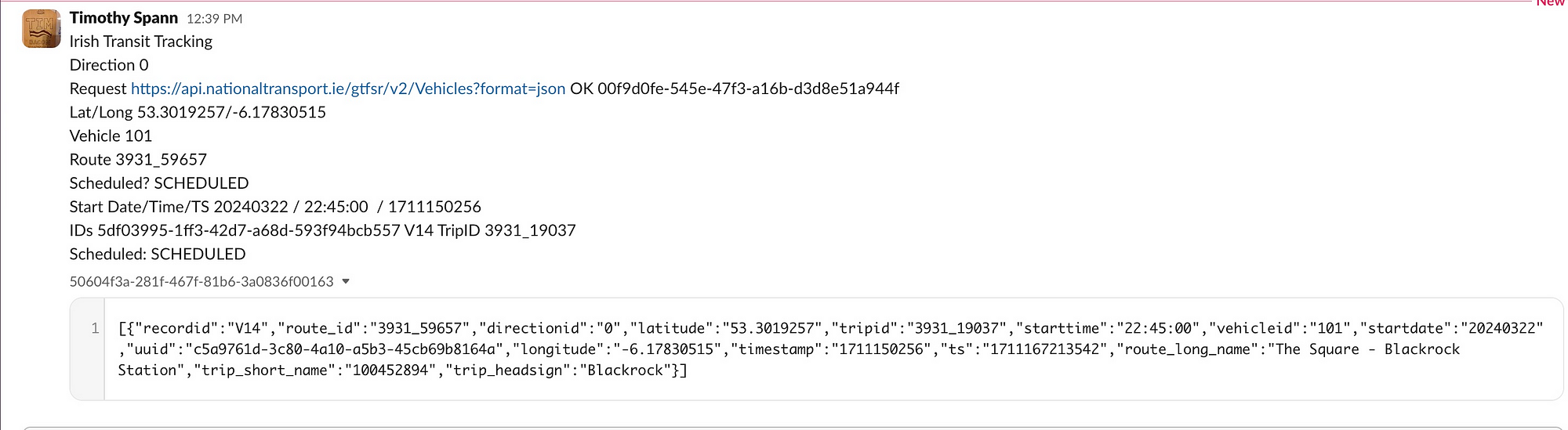
NATIONAL ROADS WEATHER STATION
Real-time data from TII's national network of 80+ weather stations. Includes air temperature, precipitation, wind speed…data.gov.ie
PUBLIC TRANSPORT DATA
Any cookies that may not be particularly necessary for the website to function and is used specifically to collect user…www.transportforireland.ie
LOOKUP DATA FROM GTFS
This document defines the format and structure of the files that comprise a GTFS dataset. The key words "MUST", "MUST…gtfs.org
stop_id
Unique ID
This document defines the format and structure of the files that comprise a GTFS dataset. The key words "MUST", "MUST…gtfs.org
Primary key (trip_id
, stop_sequence
)
This document defines the format and structure of the files that comprise a GTFS dataset. The key words "MUST", "MUST…gtfs.org
DUBLIN BIKES
RAILROAD
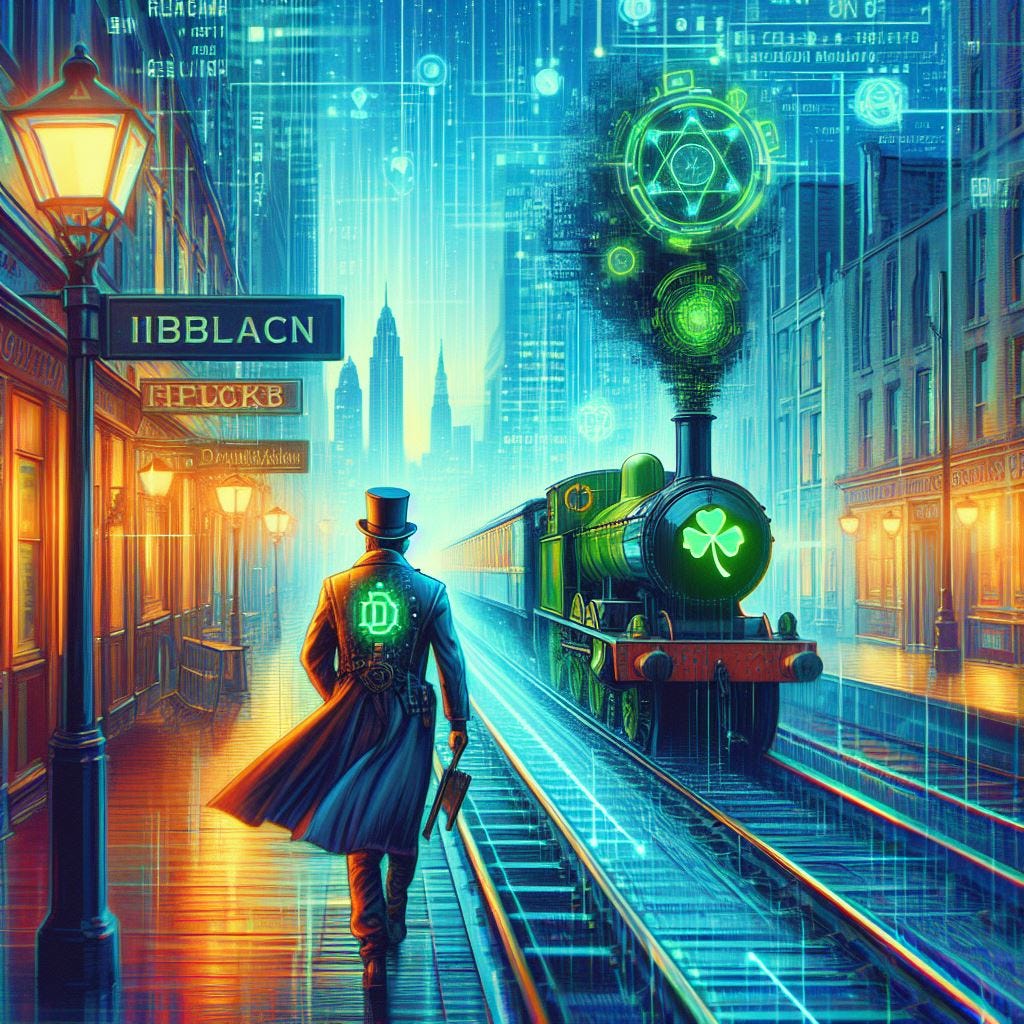
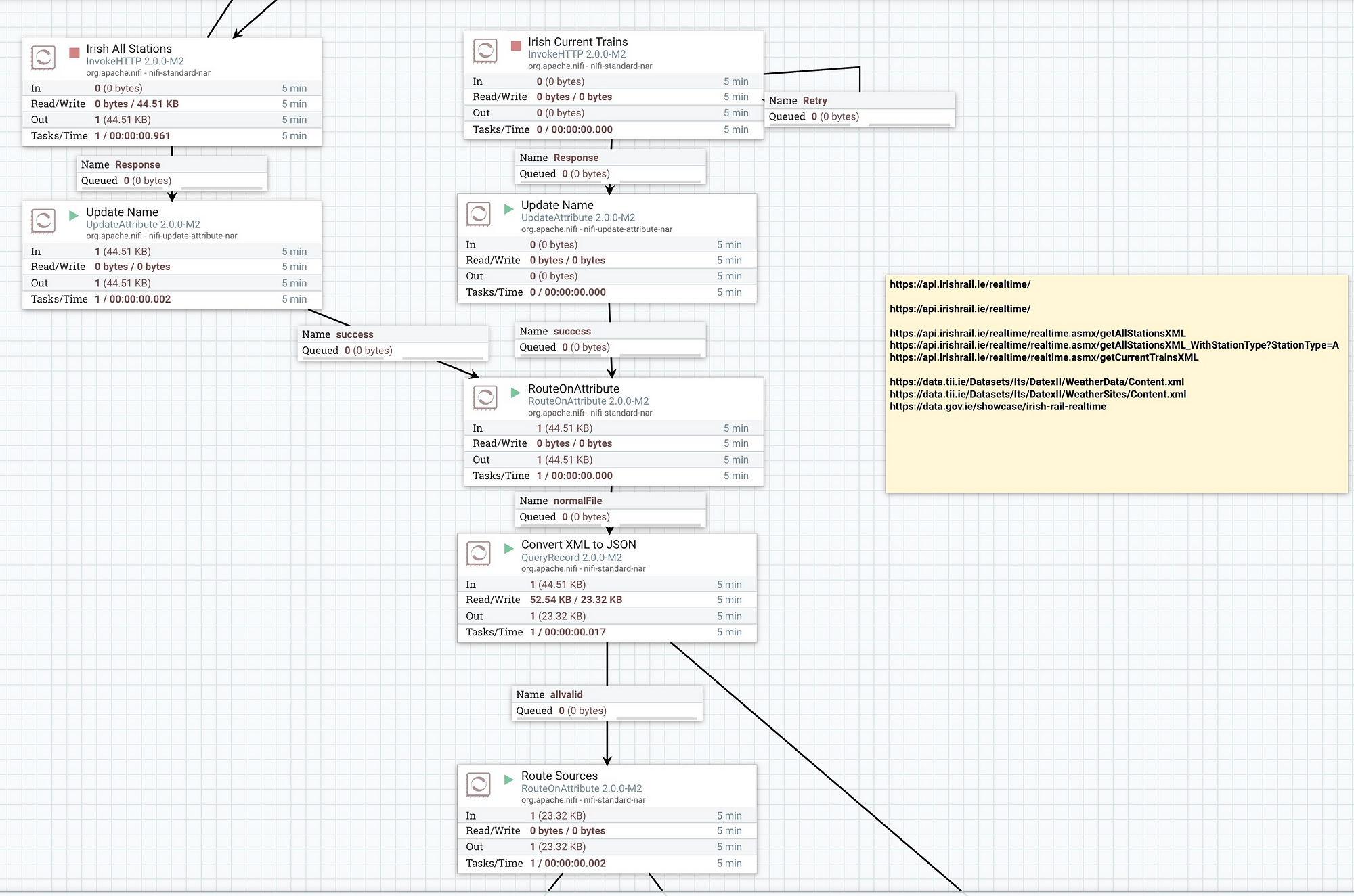
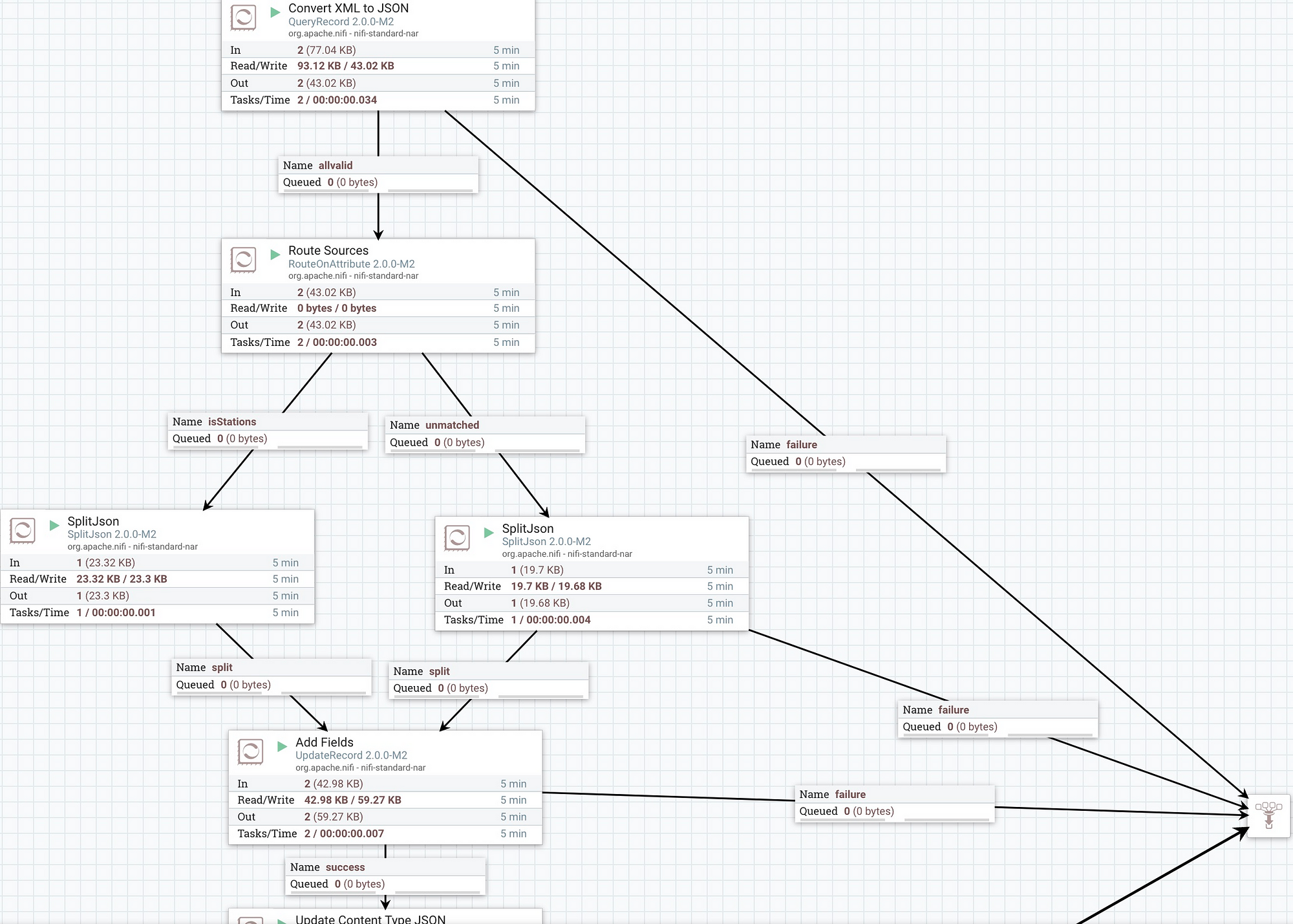
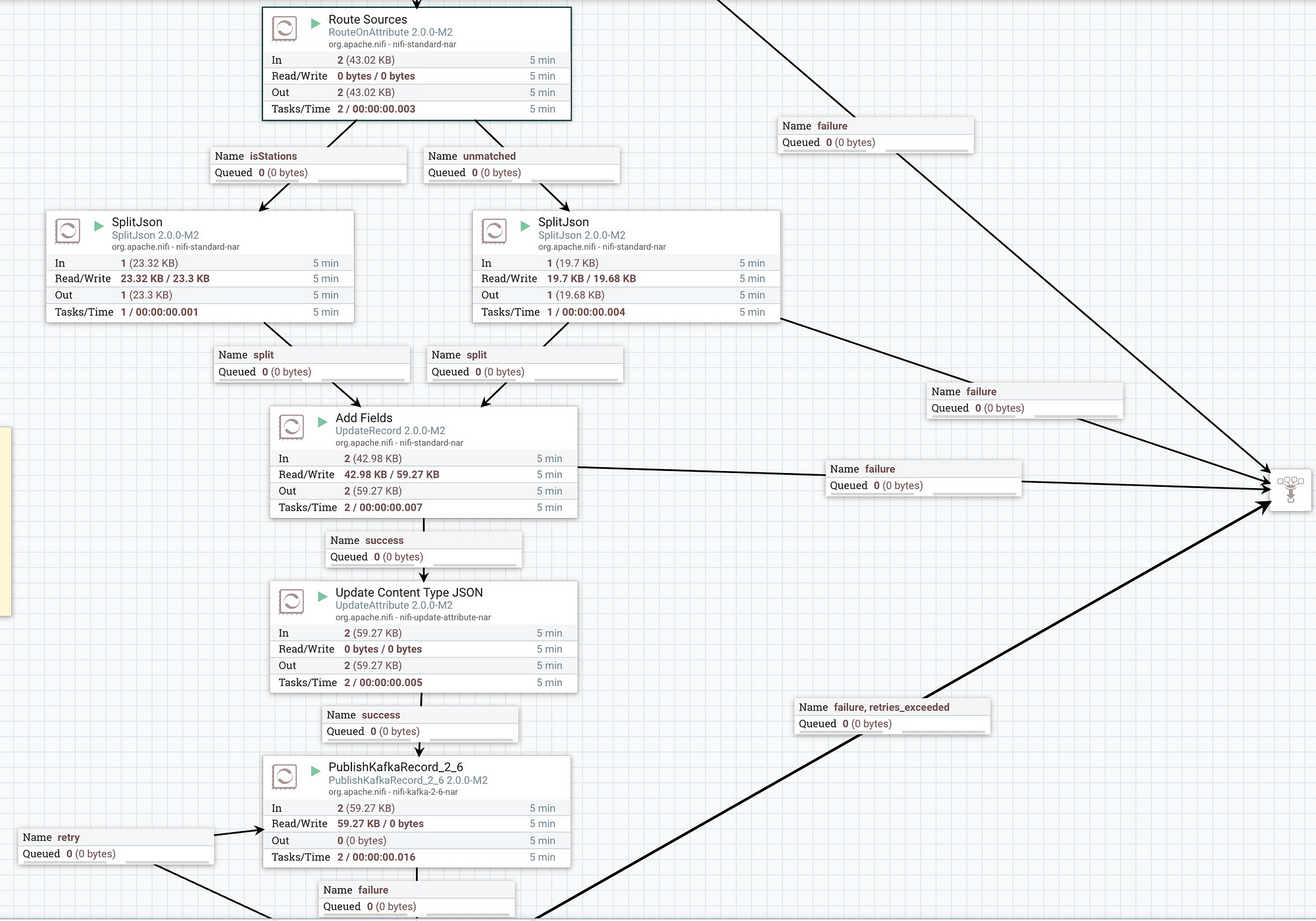
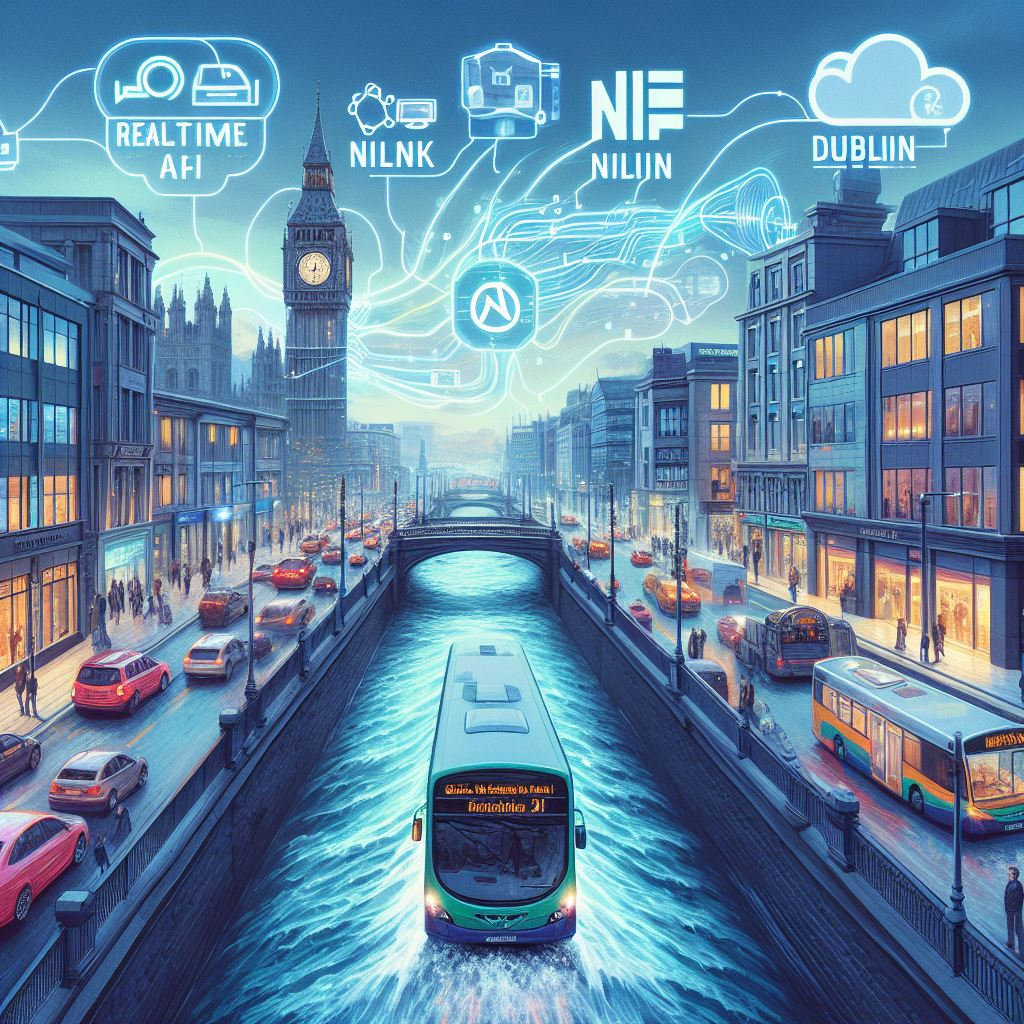
IRISH STATIONS
{"StationDesc":"Millstreet","StationAlias":null,
"StationLatitude":52.0776,"StationLongitude":-9.06973,
"StationCode":"MLSRT","StationId":24,"ts":"1711496919762",
"uuid":"f6e71a76-41cc-4a8e-8795-323c3b43d62f"}
IRISH TRAIN RECORD
{
"TrainStatus":"R","TrainLatitude":53.4169,
"TrainLongitude":-6.1512,"TrainCode":"P617",
"TrainDate":"27 Mar 2024",
"PublicMessage":"P617\\n16:02 - Drogheda to Dublin Pearse (1 mins late)\\nDeparted Portmarnock next stop Dublin Connolly",
"Direction":"Southbound","ts":"1711557932947",
"uuid":"b485cefb-67e8-482d-86ba-1ca43e0b523a"
}
IRISH STATION RECORD
{
"StationDesc":"Midleton",
"StationAlias":null,
"StationLatitude":51.9212,
"StationLongitude":-8.17579,
"StationCode":"MDLTN",
"StationId":68,
"ts":"1711558009615",
"uuid":"1f5ae394-4726-4f3e-8e53-7f50f95ae05e"
}
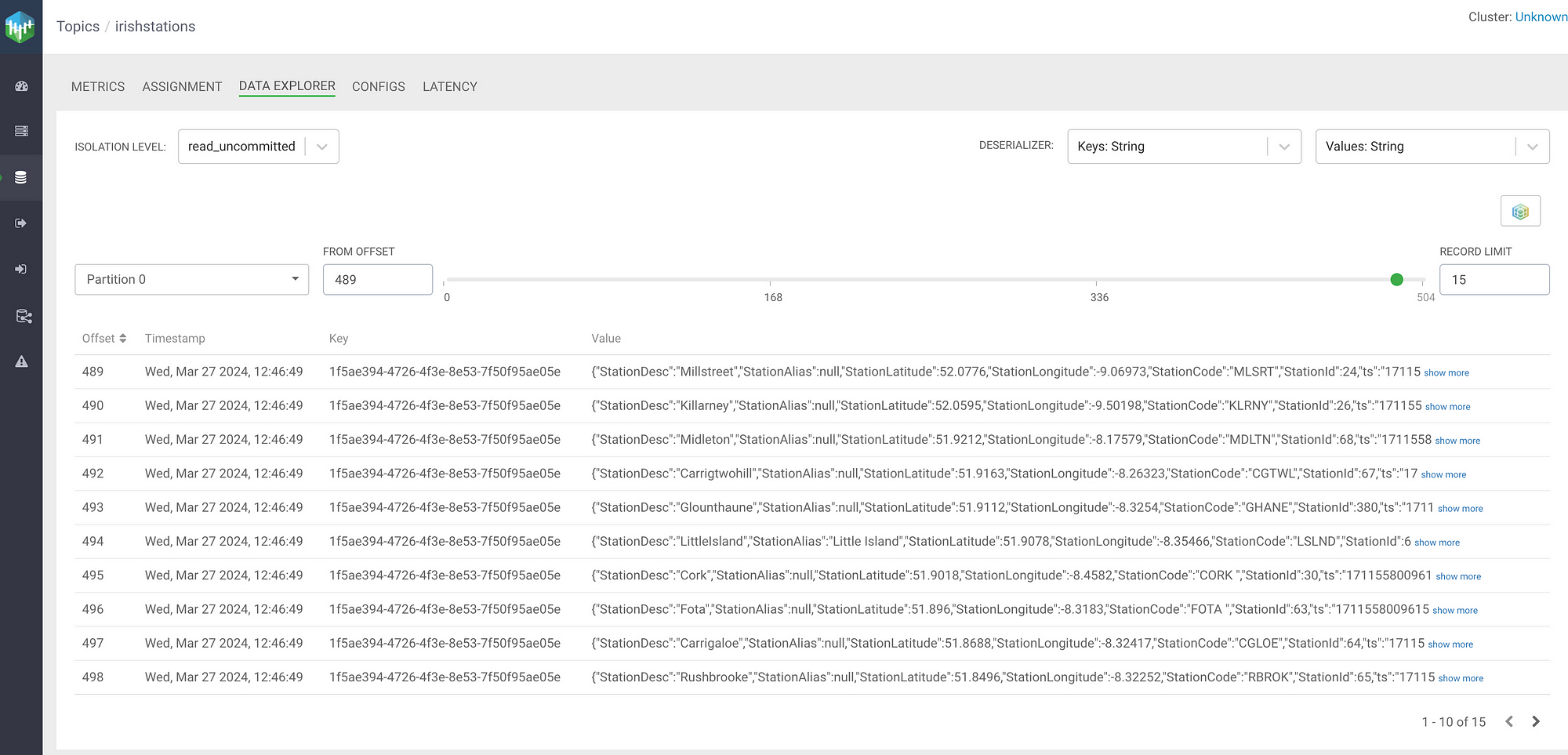
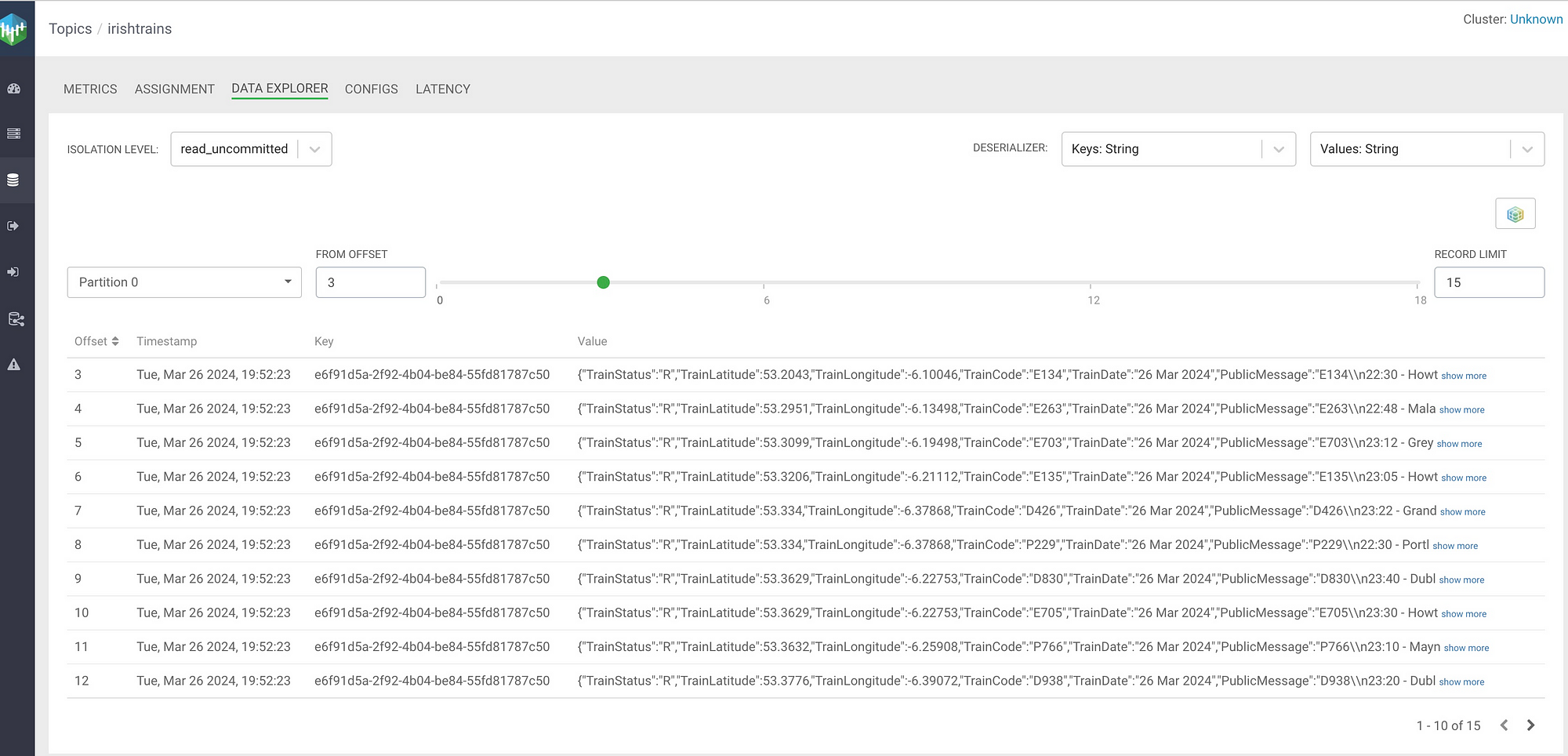
SOURCE CODE
Transit in Ireland. Contribute to tspannhw/FLaNK-IrelandTransit development by creating an account on GitHub.github.com
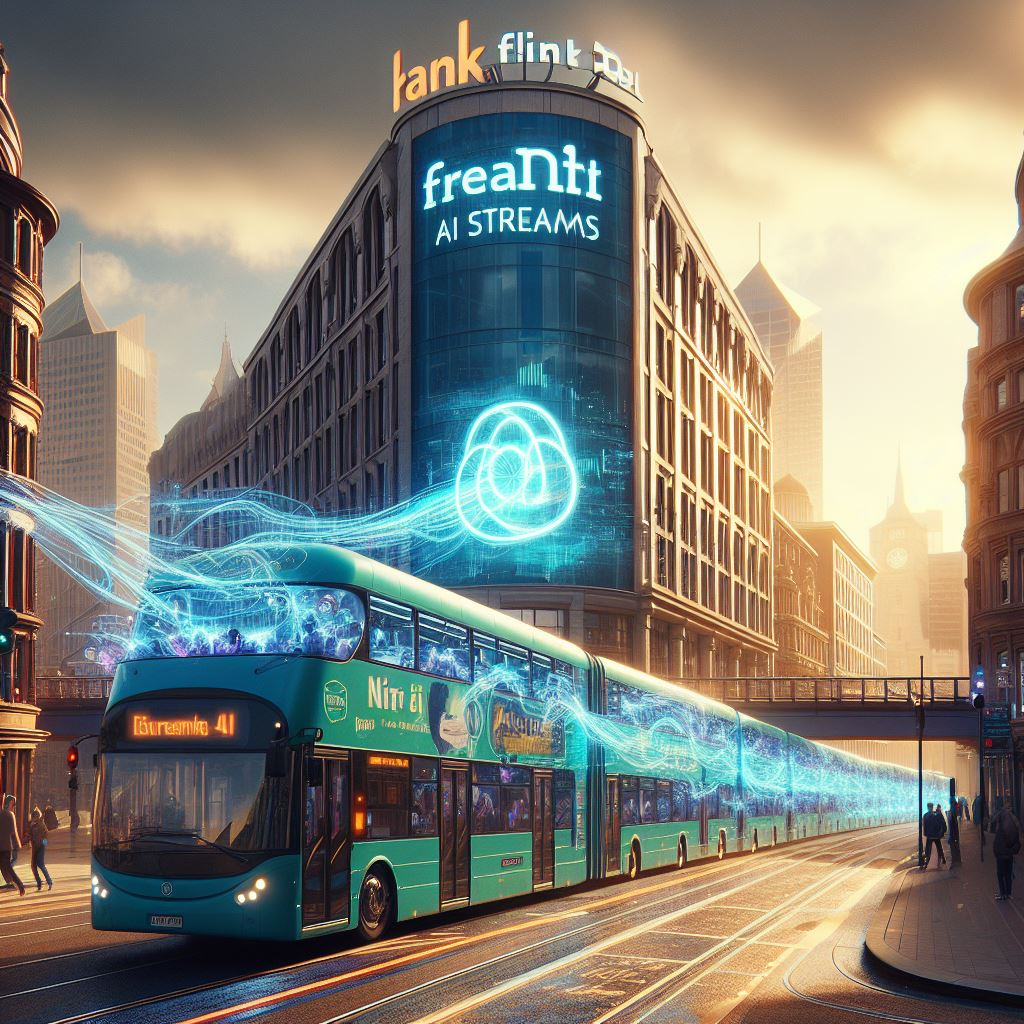
FLINK SQL KAFKA TABLE
CREATE TABLE `ssb`.`Meetups`.`irelandvehicle` (
`recordid` VARCHAR(2147483647),
`route_id` VARCHAR(2147483647),
`directionid` VARCHAR(2147483647),
`latitude` VARCHAR(2147483647),
`tripid` VARCHAR(2147483647),
`starttime` VARCHAR(2147483647),
`vehicleid` VARCHAR(2147483647),
`startdate` VARCHAR(2147483647),
`uuid` VARCHAR(2147483647),
`longitude` VARCHAR(2147483647),
`timestamp` VARCHAR(2147483647),
`ts` VARCHAR(2147483647),
`route_long_name` VARCHAR(2147483647),
`trip_short_name` VARCHAR(2147483647),
`trip_headsign` VARCHAR(2147483647),
`eventTimeStamp` TIMESTAMP(3) WITH LOCAL TIME ZONE METADATA FROM 'timestamp',
WATERMARK FOR `eventTimeStamp` AS `eventTimeStamp` - INTERVAL '3' SECOND
) WITH (
'scan.startup.mode' = 'group-offsets',
'deserialization.failure.policy' = 'ignore_and_log',
'properties.request.timeout.ms' = '120000',
'properties.auto.offset.reset' = 'earliest',
'format' = 'json',
'properties.bootstrap.servers' = 'kafka:9092',
'connector' = 'kafka',
'properties.transaction.timeout.ms' = '900000',
'topic' = 'irelandvehicle',
'properties.group.id' = 'irelandconsumersbb1'
)
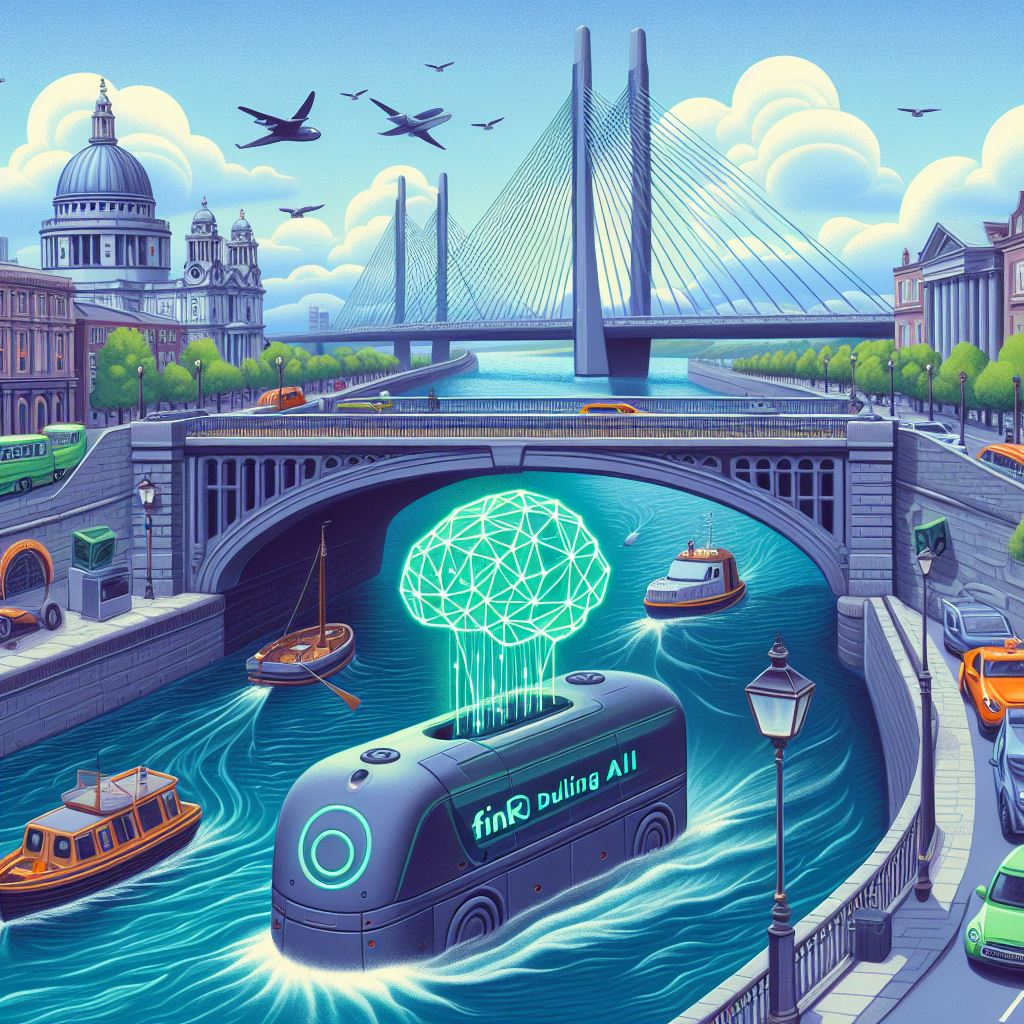
RESOURCES
We're excited to announce that Cloudera has been named the Best Medium Workplace in Ireland™, one of the Best…blog.cloudera.com
I have a plan to write a 3 part "intro" series as to how to handle your XML files.www.linkedin.com
Get actionable tips and insights about Apache NiFi, an open-source tool with a drag-and-drop interface for building…www.cloudera.com
This recipe helps you extract values from XML data in NiFiwww.projectpro.io
I created an install script (linux machines) that will install a local secure version of NiFi. It will also generate…www.silvercloudcomputing.com
EverythingApacheNiFi. Contribute to tspannhw/EverythingApacheNiFi development by creating an account on GitHub.github.com
OBJECTIVE: Provide a quick-start guide for using the Jolt language within a NiFi JoltTransform (JoltTransformJSON or…community.cloudera.com
This document defines the format and structure of the files that comprise a GTFS dataset. The key words "MUST", "MUST…gtfs.org
Hello all Within NiFi, updateAttribute processor I am trying to change an attribute called 'hive_database' based on the…community.cloudera.com
Updates the Attributes for a FlowFile by using the Attribute Expression Language and/or deletes the attributes based on…nifi.apache.org
When it comes to creating resilient data pipelines, one of the tools that comes to mind is Apache NiFi. At the heart of…medium.com
Collecting data from SQL and NoSQL systems and building a data ingestion pipeline can be a complex process, but it can…pratikbarjatya.medium.com
Canonical GTFS Validator project for schedule (static) files. - MobilityData/gtfs-validatorgithub.com
Format for exchanging realtime public transit information.developers.google.com
Community list of transit APIs, apps, datasets, research, and software :bus::star2::train::star2::steam_locomotive: …github.com
Apache NiFi, Python, Traffic, JSON, Web Camera, REST, XML, RSS, JSONmedium.com
This is the remix.medium.com
NiFi-Kafka-Flink for getting to work. Can’t we just work remote?medium.com
Event Streaming in Canada with NiFi, Kafka, Flink, PostgreSQLmedium.com
Source Code: https://github.com/tspannhw/FLaNK-EveryTransitSystemmedium.com
Contribute to google/transit development by creating an account on GitHub.github.com
Contribute to google/transit development by creating an account on GitHub.github.com
A GTFS Realtime feed lets transit agencies provide consumers with realtime information about disruptions to their…gtfs.org
View live departures. Search for and select a stop to view available departure times, with map and satellite views…www.transportforireland.ie
Open source routing engine for OpenStreetMap. Use it as Java library or standalone web server. …github.com
Open source routing engine for OpenStreetMap. Use it as Java library or standalone web server. - graphhopper/README.md…github.com
OneBusAway is a suite of open source transit information tools that enable transit agencies to provide real-time…developer.onebusaway.org
Docker installer for TheTransitClock. Contribute to TheTransitClock/transitclockDocker development by creating an…github.com
TheTransitClock real-time transit information system - TheTransitClock/transitimegithub.com
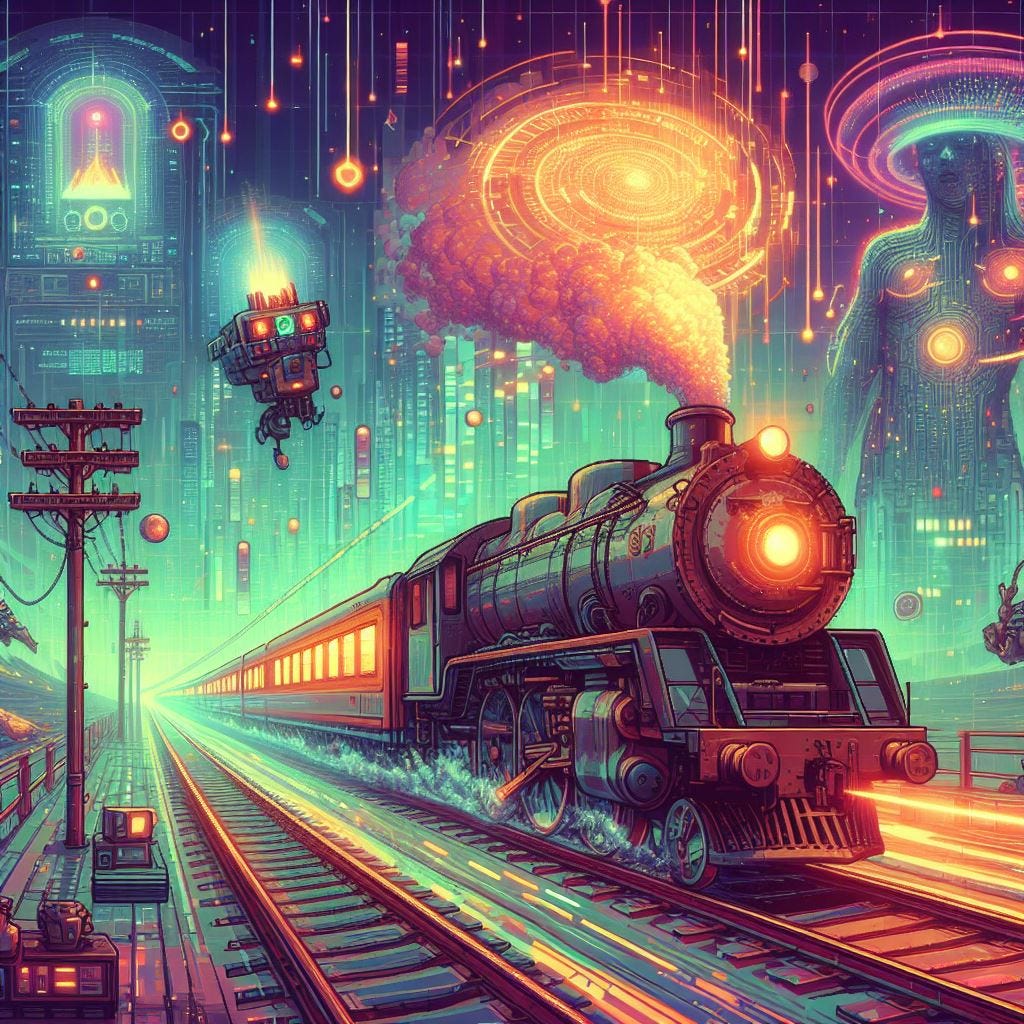