Let’s Check Our Stocks From FinnHub and Do Some Real-Time Analytics
Code: https://github.com/tspannhw/FLiPN-Py-Stocks
The easiest application to build is a simple Python application since finnhub includes the basics in their documentation. We are going to use their free WEBSOCKET interface to Trades so we can get real-time events as they happen. We will get JSON data for each trade triggered.
Python App
Python application receives websocket stream of JSON arrays and sends individual JSON messages with a JSON schema.
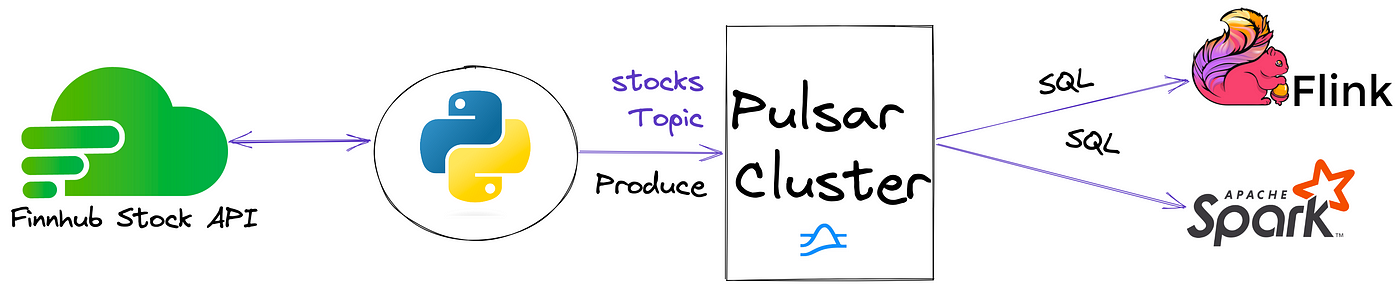
Raw Data
{"data":[{"c":["1","8","24","12"],"p":122.1,"s":"TSLA","t":1672348887195,"v":1},{"c":["1","8","24","12"],"p":122.09,"s":"TSLA","t":1672348887196,"v":4},{"c":["1","8","24","12"],"p":122.09,"s":"TSLA","t":1672348887196,"v":10},{"c":["1","8","24","12"],"p":122.1,"s":"TSLA","t":1672348887196,"v":1},{"c":["1","8","24","12"],"p":122.1,"s":"TSLA","t":1672348887196,"v":2},{"c":["1","8","24","12"],"p":122.1,"s":"TSLA","t":1672348887196,"v":10},{"c":["1","8","24","12"],"p":122.1,"s":"TSLA","t":1672348887198,"v":79},{"c":["1","24","12"],"p":129.58,"s":"AAPL","t":1672348887666,"v":1},{"c":["1","24","12"],"p":129.575,"s":"AAPL","t":1672348887785,"v":1}],"type":"trade"}
{"c":["1","8","24","12"],"p":122.1,"s":"TSLA","t":1672348887195,"v":1}
Data Description
data
List of trades or price updates.s
Symbol.p
Last price.t
UNIX milliseconds timestamp.v
Volume.c
List of trade conditions. A comprehensive list of trade conditions code can be found here
Let’s Build a Schema for our JSON data. Once we have a class definied for it in Python, we can send that to an Apache Pulsar cluster and it will generate the first version of our schema for us. When we have a schema it lets us treat that data as a table in Trino, Spark SQL and Flink SQL. So this is awesome.
By defining our data and making it fully structured with a schema even though it is still semi-structured JSON, it makes it very easy to work with. We know what we are getting. This will make it easier to stream into Apache Pinot, Apache Iceberg, Delta Lake or another analytics system.
class Stock (Record):
symbol = String()
ts = Float()
currentts = Float()
volume = Float()
price = Float()
tradeconditions = String()
uuid = String()
We then connect to our Pulsar cluster, very easy in Python.
client = pulsar.Client(‘pulsar://localhost:6650’)
producer = client.create_producer(topic=’persistent://public/default/stocks’ ,schema=JsonSchema(Stock),properties={“producer-name”: “py-stocks”,”producer-id”: “pystocks1” })
If we have never used this topic before, Pulsar will create it for you. For best practices, build your tenant, namespace and topic before your application while you are defining schemas and data contracts.
For more information on the Python interface for Pulsar, check out this link.
NEWBIE HINT:
For a free cluster and training, check out this training academy.
Example Python Projects
For all the real newbies, here is the real getting started.
Consume Pulsar Data
bin/pulsar-client consume "persistent://public/default/stocks" -s stocks-reader -n 0
----- got message -----
key:[20221230191756_42a4752d-5f66-4245-8153-a5ec8478f738], properties:[], content:{
"symbol": "AAPL",
"ts": 1672427874976.0,
"currentts": 20221230191756.0,
"volume": 10.0,
"price": 128.055,
"tradeconditions": "1 12",
"uuid": "20221230191756_42a4752d-5f66-4245-8153-a5ec8478f738"
}
----- got message -----
key:[20221230191756_a560a594-7c12-42e7-a76d-6650a48533e0], properties:[], content:{
"symbol": "TSLA",
"ts": 1672427874974.0,
"currentts": 20221230191756.0,
"volume": 100.0,
"price": 120.94,
"tradeconditions": "",
"uuid": "20221230191756_a560a594-7c12-42e7-a76d-6650a48533e0"
}