Connecting Apache NiFi to Apache Atlas For Data Governance At Scale in Streaming
Once connected you can see NiFi and Kafka flowing to Atlas.
You must add Atlas Report to NiFi cluster.
Add a ReportLineageToAtlas under Controller Settings / Reporting Tasks
You must add URL for Atlas, Authentication method and if basic, username/password.
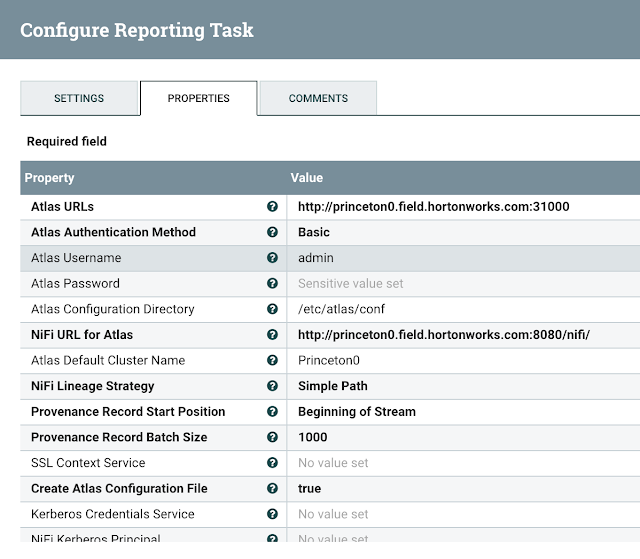
You need to set Atlas Configuration directory, NiFi URL to use, Lineage Strategy - Complete Path
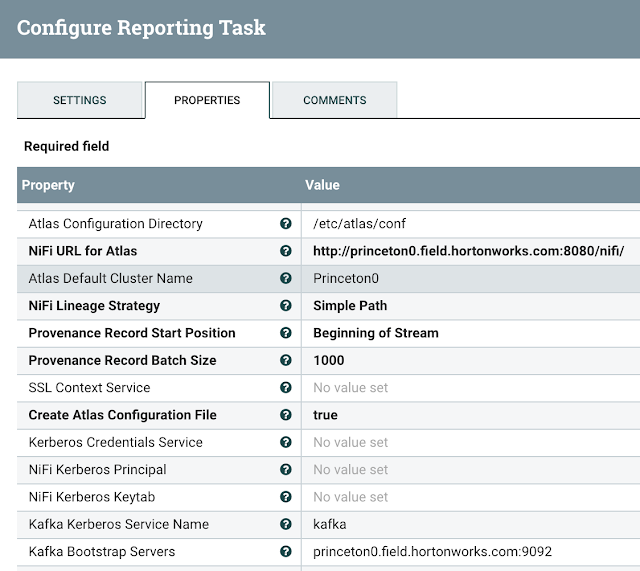
Another example with an AWS hosted NiFi and Atlas:
IMPORTANT NOTE: Keep your Atlas Default Cluster Name consistent with other applications for Cloudera clusters, usually the name cm is a great option or default.
You can now see the lineage state:
Configure Atlas to Be Enabled and Have Kafka
Have Atlas Service enabled in NiFi configuration
Example Configuration
You must have access to Atlas Application Properties.
/etc/atlas/conf
atlas-application.properties
#Generated by Apache NiFi ReportLineageToAtlas ReportingTask at 2020-02-21T17:18:28.493Z
#Fri Feb 21 17:18:28 UTC 2020
atlas.kafka.bootstrap.servers=princeton0.field.hortonworks.com\:9092
atlas.enableTLS=false
atlas.kafka.client.id=ReportLineageToAtlas.687a48e2-0170-1000-0000-00000a0de4ea
atlas.cluster.name=Princeton0
atlas.kafka.security.protocol=PLAINTEXT
atlas-server.properties
princeton0.field.hortonworks.com:atlas.authentication.method.kerberos=false
princeton0.field.hortonworks.com:atlas.enableTLS=false
princeton0.field.hortonworks.com:atlas.kafka.zookeeper.connection.timeout.ms=30000
princeton0.field.hortonworks.com:atlas.kafka.zookeeper.session.timeout.ms=60000
princeton0.field.hortonworks.com:atlas.kafka.zookeeper.sync.time.ms=20
princeton0.field.hortonworks.com:atlas.server.bind.address=0.0.0.0
princeton0.field.hortonworks.com:atlas.server.http.port=31000
princeton0.field.hortonworks.com:atlas.server.https.port=31443
Running Atlas
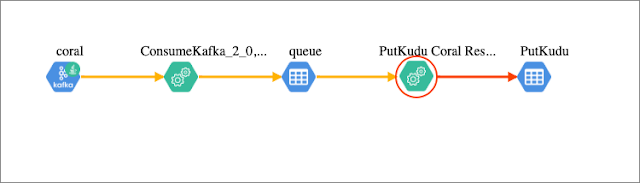
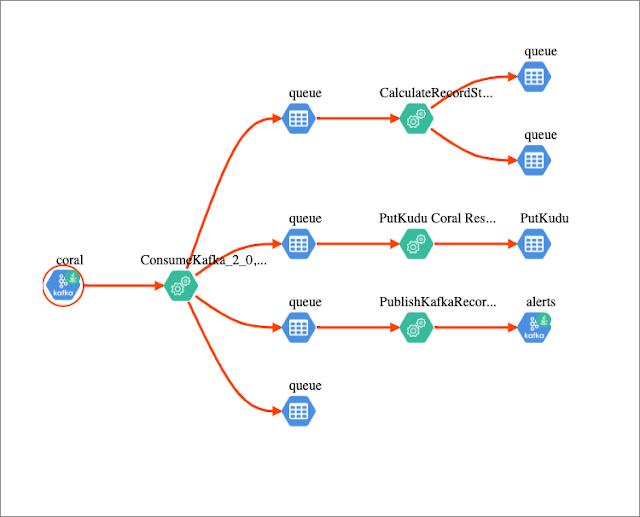
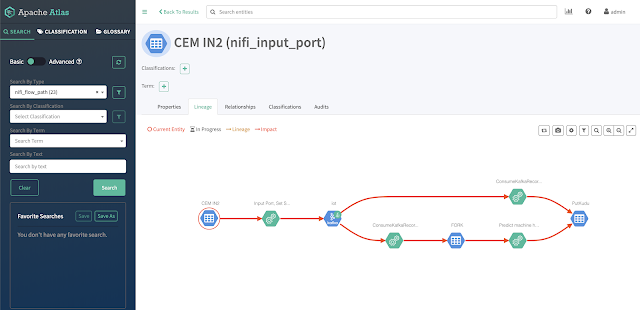
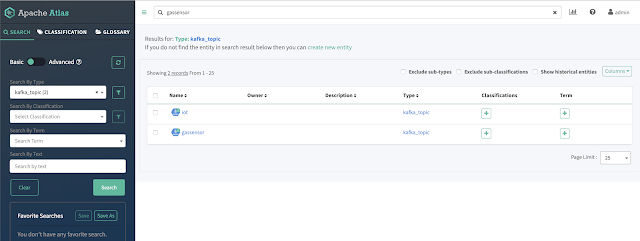
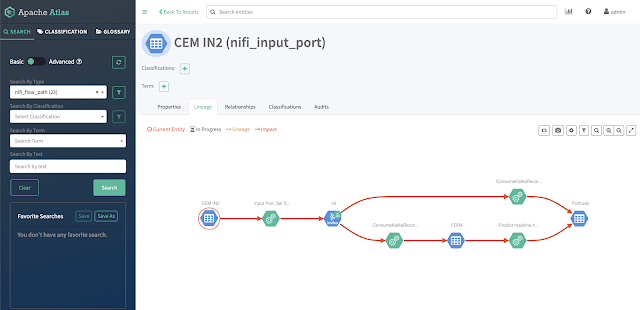
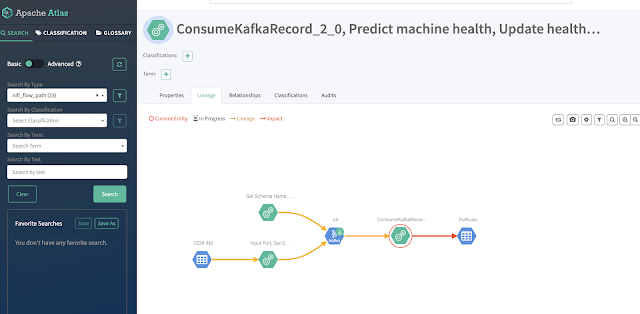
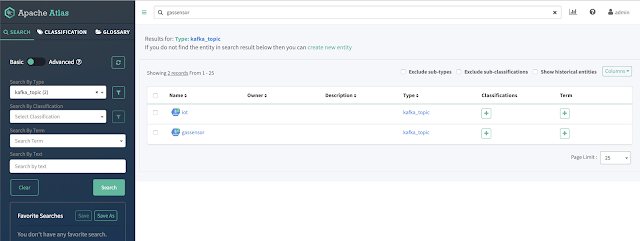
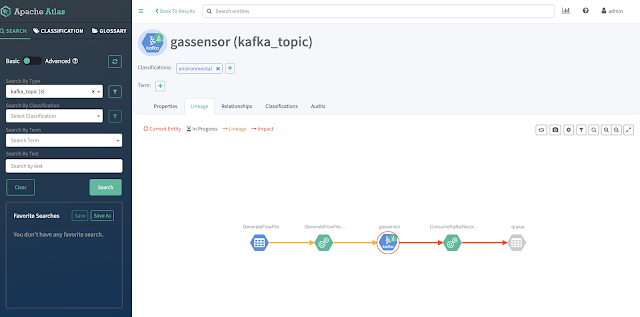
See:
- https://atlas.apache.org/#/
- https://medium.com/@kandalkarbhushan/integrating-nifi-with-atlas-in-secured-environment-c1b0f3cb7318
- https://docs.cloudera.com/HDPDocuments/HDF3/HDF-3.1.1/bk_installing-hdf-and-hdp/content/nifi-atlas.html
- https://docs.cloudera.com/HDPDocuments/HDF3/HDF-3.4.1.1/installing-hdf-and-hdp-ppc/content/configure_nifi_for_atlas_integration.html
- https://docs.cloudera.com/runtime/7.0.2/cdp-governance-overview/topics/atlas-overview.html
- https://www.datainmotion.dev/2020/02/apache-atlas-for-monitoring-edge2ai-iot.html
- https://docs.cloudera.com/cloudera-manager/7.0.3/reference/topics/cm_props_cr703_atlas.html